The Future of Trading: AI in Stocks and Forex
The blog post explores the potential of AI in the trading of stocks and forex, highlighting its fascinating application in the trading industry.
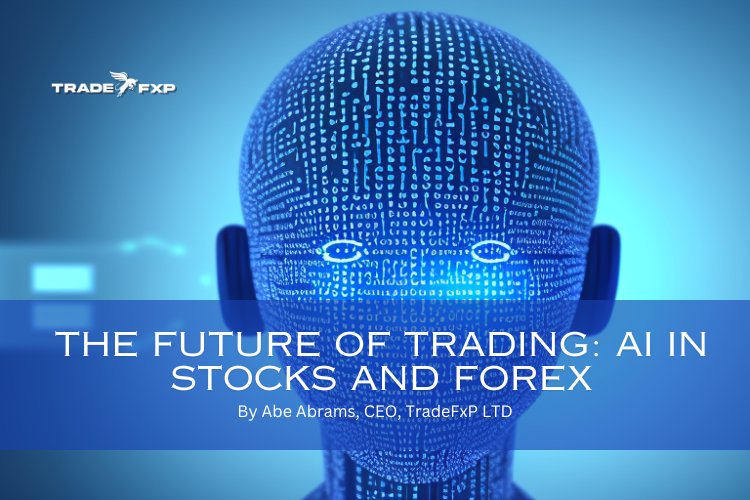
You can download this article as a PDF at the bottom of this page
The Future of Trading: AI in Stocks and Forex
By Abe Abrams, CEO, TradeFxP LTD
Book Overview
In "The Future of Trading: AI in Stocks and Forex," we delve into the exciting world of using artificial intelligence (AI) in the realm of trading. This book explores how AI is revolutionising the way stocks and forex are traded and the potential it holds for the future. We examine the pros and cons of incorporating AI into trading strategies, providing readers with a comprehensive understanding of the benefits and challenges that come with this technology.
Throughout the book, we discuss the various ways in which AI is being utilized in the trading industry. From algorithmic trading to predictive analytics, we explore how AI can analyse vast amounts of data and make informed trading decisions in real-time. We also examine the potential risks and limitations of relying on AI, such as the possibility of algorithmic biases and the need for human oversight.
"The Future of Trading: AI in Stocks and Forex" aims to equip traders, investors, and enthusiasts with the knowledge they need to navigate the evolving landscape of AI in trading. Whether you are a seasoned trader or just starting out, this book provides valuable insights into the future of trading and how AI can be leveraged to enhance profitability and efficiency. Join us on this journey as we explore the potential of AI in stocks and forex trading.
Introduction to AI in Trading
Understanding artificial intelligence
Artificial intelligence (AI) is a rapidly evolving field that has the potential to revolutionise various industries, including trading stocks and forex. AI refers to the development of computer systems that can perform tasks that typically require human intelligence, such as decision-making, problem-solving, and learning from experience. In the context of trading, AI algorithms are designed to analyse vast amounts of data, identify patterns, and make predictions to inform trading decisions.
AI in trading is not a new concept, but recent advancements in technology, such as increased computing power and the availability of big data, have significantly enhanced its capabilities. AI algorithms can process and analyse large volumes of financial data in real time, enabling traders to make more informed and data-driven decisions. This has the potential to improve trading strategies, increase profitability, and reduce risks.
There are several key components of AI that are relevant to trading. Machine learning, a subset of AI, involves the development of algorithms that can learn from data and improve their performance over time without being explicitly programmed. Deep learning, another subset of AI, focuses on training neural networks with multiple layers to recognise complex patterns and make accurate predictions. Natural Language Processing (NLP) enables computers to understand and interpret human language, which is particularly useful for analysing news articles, social media sentiment, and other textual data relevant to trading. Lastly, reinforcement learning involves training algorithms to make decisions based on trial and error and receiving feedback and rewards for their actions.
The application of AI to trading stocks and forex offers several advantages. Firstly, AI algorithms can process vast amounts of data much faster than humans, enabling traders to make quicker and more informed decisions. This can be particularly beneficial in high-frequency trading, where split-second decisions can make a significant difference in profitability. Secondly, AI algorithms can identify patterns and trends in the market that may not be apparent to human traders. By analysing historical data and real-time market conditions, AI can detect subtle correlations and make predictions with a higher degree of accuracy. This can help traders identify profitable opportunities and optimise their trading strategies. Thirdly, AI can reduce human bias and emotions in trading decisions. Emotions such as fear and greed can often cloud judgement and lead to irrational trading decisions. AI algorithms, on the other hand, are not influenced by emotions and can make objective decisions based on data and predefined rules.
Despite the numerous benefits, there are also limitations and challenges associated with the use of AI in trading. Firstly, AI algorithms heavily rely on historical data to make predictions about the future. However, financial markets are dynamic and subject to various external factors, such as economic events, political developments, and natural disasters, which can significantly impact market conditions. Therefore, there is always a degree of uncertainty associated with AI predictions. Secondly, AI algorithms can be complex and require significant computational resources. Implementing and maintaining AI systems can be costly, especially for smaller traders or firms. Additionally, AI algorithms are not infallible and can make incorrect predictions or decisions. It is crucial for traders to understand the limitations of AI and not solely rely on its recommendations.
Another important consideration is the ethical implications of using AI in trading. AI algorithms can potentially exacerbate market volatility and contribute to market manipulation if not properly regulated. There is a need for transparency and accountability in AI trading systems to ensure fair and ethical practices. Additionally, there is a concern that AI algorithms may perpetuate biases present in the data they are trained on, leading to unfair outcomes. It is essential to address these ethical concerns and develop frameworks that promote fairness and prevent discriminatory practices in AI trading.
AI has the potential to revolutionise trading stocks and forex by enabling faster and more informed decision-making, identifying patterns and trends, and reducing human bias. However, it is important to understand the limitations and challenges associated with AI, such as the reliance on historical data, computational requirements, and ethical considerations. As technology continues to advance, it is crucial for traders and regulators to adapt and develop frameworks that ensure the responsible and ethical use of AI in trading.
Overview of Trading Stocks and Forex
Trading stocks and forex are two popular investment markets that attract millions of traders worldwide. In this section, we will provide an overview of these markets, their characteristics, and how they operate.
Stocks Market
The stock market is a marketplace where individuals and institutions can buy and sell shares of publicly traded companies. It is a vital component of the global financial system and plays a crucial role in the economy. The stock market provides companies with a platform to raise capital by selling shares, while investors have the opportunity to participate in the growth and profitability of these companies.
Stocks represent ownership in a company and are typically issued in the form of shares. When investors buy shares, they become partial owners of the company and have the potential to benefit from its success. The value of stocks fluctuates based on various factors such as company performance, market conditions, economic indicators, and investor sentiment.
Trading stocks involves analysing company financials, market trends, and other relevant information to make informed investment decisions. Traders can choose to buy stocks with the intention of holding them for the long term (known as investing) or for short-term gains (known as trading).
Forex Market
The forex market, also known as the foreign exchange market, is the largest and most liquid financial market globally. It involves the buying and selling of currencies from different countries. Unlike the stock market, which operates through centralised exchanges, forex trading takes place over-the-counter (OTC) through a decentralised network of banks, financial institutions, and individual traders.
The forex market facilitates international trade and investment by enabling the conversion of one currency into another. It plays a crucial role in determining exchange rates between currencies, which have a significant impact on global trade and economic stability.
Forex trading involves speculating on the price movements of currency pairs. Traders aim to profit from fluctuations in exchange rates by buying a currency at a lower price and selling it at a higher price, or vice versa. The forex market operates 24 hours a day, five days a week, allowing traders to participate in trading activities across different time zones.
Similarities and differences
While both stock and forex markets involve buying and selling financial instruments, there are several key differences between them.
Market Size: The forex market is significantly larger than the stock market in terms of daily trading volume. The immense liquidity of the forex market allows for seamless execution of trades, even for large transactions.
Market Structure: The stock market operates through centralised exchanges, where buyers and sellers trade shares of publicly listed companies. In contrast, the forex market is decentralised, with trading occurring directly between participants.
Influence Factors: Company-specific factors like earnings reports, management choices, and industry trends influence stock prices. On the other hand, central bank policies, geopolitical events, and macroeconomic factors all affect forex prices.
Trading Hours: The stock market has fixed trading hours, typically from morning to afternoon, based on the local time zone. In contrast, the forex market operates 24 hours a day, allowing traders to engage in trading activities at any time.
Leverage and Margin: Forex trading offers higher leverage and margin requirements compared to stock trading. This allows traders to control larger positions with a smaller amount of capital, potentially amplifying both profits and losses.
Market Accessibility: The stock market is accessible to both individual and institutional investors. In contrast, the forex market is more accessible to individual traders due to lower entry barriers, such as lower capital requirements and the availability of online trading platforms.
Understanding the basics of trading stocks and forex is essential before delving into the role of artificial intelligence (AI) in these markets. While stocks represent ownership in companies, forex trading involves speculating on currency price movements. Both markets have their own unique characteristics, influencing factors, and trading dynamics. In the following sections, we will explore how AI is revolutionising trading in these markets, enabling traders to make more informed decisions and potentially enhance their trading strategies.
The role of AI in trading
Artificial intelligence (AI) has revolutionised various industries, and the world of trading is no exception. In recent years, AI has emerged as a powerful tool for traders in both the stock and forex markets. By leveraging advanced algorithms and machine learning techniques, AI has the potential to enhance decision-making, automate trading processes, and improve overall profitability. This section explores the role of AI in trading, highlighting its key benefits and limitations.
Enhancing Decision-Making
One of the primary roles of AI in trading is to enhance decision-making capabilities. Traditional trading strategies often rely on human intuition and analysis, which can be subjective and prone to bias. AI, on the other hand, can process vast amounts of data, identify patterns, and make data-driven predictions. By analysing historical market data, news sentiment, and other relevant factors, AI algorithms can generate insights and recommendations that assist traders in making informed decisions.
AI-powered trading systems can quickly analyse multiple indicators and factors simultaneously, providing traders with a comprehensive view of the market. This enables them to identify potential trading opportunities, predict market trends, and execute trades with greater precision and speed. By reducing human error and emotional biases, AI can help traders optimize their strategies and improve overall performance.
Automation and efficiency
Another crucial role of AI in trading is automation. AI algorithms can be programmed to execute trades automatically based on predefined rules and parameters. This automation eliminates the need for manual intervention and allows traders to take advantage of market opportunities 24/7. By continuously monitoring market conditions and reacting to changes in real-time, AI-powered trading systems can execute trades with exceptional speed and accuracy.
Furthermore, AI can automate various trading tasks, such as data collection, analysis, and risk management. By automating these processes, traders can save time and focus on higher-level decision-making. AI algorithms can also adapt and learn from market conditions, continuously improving their performance over time. This adaptability and efficiency make AI an invaluable tool for traders seeking to optimise their trading strategies and maximise profits.
Risk Management and Mitigation
AI plays a crucial role in risk management and mitigation in trading. By analysing historical market data and identifying patterns, AI algorithms can assess the risk associated with specific trades or investment strategies. This allows traders to make more informed decisions and manage their risk exposure effectively.
AI can also help traders implement risk management techniques such as stop-loss orders and position sizing. By setting predefined rules and parameters, AI algorithms can automatically execute risk management strategies, ensuring that losses are minimised and profits are protected. This proactive approach to risk management can significantly enhance the overall stability and profitability of trading operations.
Market analysis and prediction
AI excels at analysing vast amounts of data and extracting meaningful insights. In trading, AI algorithms can analyse historical market data, news articles, social media sentiment, and other relevant information to identify patterns and predict market trends. By leveraging machine learning techniques, AI can continuously learn from new data and adapt its predictions accordingly.
AI-powered trading systems can generate real-time market analysis and predictions, providing traders with valuable information to make informed decisions. These predictions can range from short-term price movements to long-term market trends. By accurately predicting market movements, traders can capitalise on opportunities and adjust their strategies accordingly.
Limitations of AI in Trading
While AI offers numerous benefits in trading, it is essential to acknowledge its limitations. AI algorithms heavily rely on historical data and patterns, which means they may struggle to adapt to unforeseen events or market conditions. Sudden market shocks, unexpected news, or geopolitical events can disrupt the accuracy of AI predictions.
Moreover, AI algorithms are only as good as the data they are trained on. If the training data is biased or incomplete, the AI system may produce inaccurate or biased predictions. It is crucial for traders to ensure that the AI algorithms are trained on high-quality and diverse datasets to minimise these biases.
Additionally, AI algorithms can be complex and challenging to interpret. The lack of transparency and explainability in AI decision-making processes can make it difficult for traders to understand why certain recommendations or predictions are made. This lack of interpretability can hinder trust and the adoption of AI systems in trading.
AI has a significant role to play in the future of trading stocks and forex. It enhances decision-making, automates trading processes, improves risk management, and provides valuable market analysis and predictions. However, it is essential to be aware of the limitations of AI, such as its reliance on historical data, potential biases, and lack of transparency. By understanding these factors, traders can effectively harness the power of AI while mitigating its limitations.
Benefits and Limitations of AI in Trading
Artificial intelligence (AI) has revolutionised various industries, and the world of trading is no exception. The integration of AI in stock and forex trading has brought about numerous benefits, but it also comes with its own set of limitations. In this section, we will explore the advantages and drawbacks of using AI in trading.
Benefits of AI in Trading
Data Analysis and Processing: AI algorithms have the ability to analyse vast amounts of data in real-time, enabling traders to make more informed decisions. By processing historical and real-time market data, AI can identify patterns, trends, and anomalies that may not be easily detectable by human traders. This allows for more accurate predictions and better trading strategies.
Automation and Efficiency: AI-powered trading systems can automate various tasks, such as data collection, analysis, and execution of trades. This reduces the need for manual intervention and minimises the risk of human error. AI can also execute trades at high speeds, enabling traders to take advantage of fleeting market opportunities and improve overall efficiency.
Improved Decision Making: AI algorithms can process and analyse vast amounts of data within seconds, providing traders with valuable insights and recommendations. By leveraging machine learning and deep learning techniques, AI can identify profitable trading opportunities and optimise trading strategies based on historical data and market conditions. This leads to more informed and data-driven decision-making.
Risk Management: AI can play a crucial role in risk management by analysing market data and identifying potential risks and vulnerabilities. By continuously monitoring market conditions and analysing various risk factors, AI systems can provide real-time risk assessments and help traders make more informed decisions. This can help minimise losses and protect investments.
Emotionless Trading: One of the significant advantages of AI in trading is its ability to eliminate emotional biases. Human traders are often influenced by emotions such as fear, greed, and overconfidence, which can lead to irrational decision-making. AI, on the other hand, operates based on predefined algorithms and rules, devoid of emotions. This can lead to more disciplined and objective trading strategies.
24/7 Market Monitoring: AI-powered trading systems can operate round the clock, continuously monitoring market conditions and executing trades based on predefined rules. This eliminates the need for human traders to be constantly present and allows for trading opportunities to be capitalised upon even during non-trading hours. This can be particularly advantageous in the global forex market, which operates 24 hours a day.
Limitations of AI in Trading
Reliance on Historical Data: AI algorithms heavily rely on historical data to make predictions and decisions. While historical data can provide valuable insights, it may not always accurately reflect future market conditions. Sudden market changes, unexpected events, or anomalies can challenge the effectiveness of AI models that are solely based on historical data.
Complexity and Lack of Transparency: AI algorithms can be highly complex, making it difficult for traders to understand and interpret their decision-making process. This lack of transparency can be a concern, especially when it comes to regulatory compliance and ethical considerations. Traders may find it challenging to explain the rationale behind AI-driven trading decisions.
Over-Optimisation and Overfitting: AI models can be prone to over-optimisation and overfitting, where the model becomes too closely aligned with historical data and fails to generalise well to new market conditions. This can lead to poor performance and inaccurate predictions when faced with unseen data. Traders need to carefully validate and test AI models to ensure their robustness and reliability.
Dependency on Data Quality: The accuracy and reliability of AI models are highly dependent on the quality and integrity of the data used for training. If the data is incomplete, inaccurate, or biased, it can lead to flawed predictions and unreliable trading strategies. Traders need to ensure the quality and relevance of the data used to train AI models.
Technological Limitations: AI systems require significant computational power and resources to operate effectively. Implementing and maintaining AI infrastructure can be costly and may require specialised technical expertise. Additionally, AI models may face limitations in handling certain market conditions or complex trading scenarios, which can impact their performance.
Market Manipulation and Black Swan Events: AI algorithms can be vulnerable to market manipulation and unexpected events, commonly known as black swan events. Manipulative trading practices or sudden market shocks can disrupt AI models and lead to significant losses. Traders need to be aware of these risks and implement appropriate risk management strategies.
The integration of AI in stock and forex trading offers numerous benefits, including enhanced data analysis, automation, improved decision-making, risk management, emotionless trading, and 24/7 market monitoring. However, it also comes with limitations such as reliance on historical data, complexity, lack of transparency, over-optimisation, dependency on data quality, technological limitations, and vulnerability to market manipulation and black swan events. Traders need to carefully consider these factors and strike a balance between leveraging AI's capabilities and mitigating its limitations to maximise its potential in trading.
AI Techniques for Stock and Forex Trading
Machine learning in trading
Machine learning is a subset of artificial intelligence that focuses on the development of algorithms and models that can learn from and make predictions or decisions based on data. In the context of trading stocks and forex, machine learning has emerged as a powerful tool for analysing vast amounts of financial data and generating insights to inform trading strategies.
Understanding machine learning
Machine learning algorithms are designed to automatically learn and improve from experience without being explicitly programmed. They can identify patterns, relationships, and trends in data that may not be apparent to human traders. By leveraging historical market data, machine learning models can uncover hidden insights and make predictions about future market movements.
There are several types of machine learning algorithms commonly used in trading:
Supervised Learning: In supervised learning, the algorithm is trained on labelled data, where the input features and the corresponding output values are known. The algorithm learns to map the input features to the output values and can then make predictions on new, unseen data.
Unsupervised Learning: Unsupervised learning algorithms are used when the data is unlabelled or when the goal is to discover hidden patterns or groupings within the data. These algorithms can identify clusters, anomalies, or other structures in the data without any prior knowledge.
Reinforcement Learning: Reinforcement learning involves training an algorithm to make a sequence of decisions in an environment to maximise a reward signal. In the context of trading, reinforcement learning can be used to develop trading strategies that optimise long-term profitability.
Applications of Machine Learning in Trading
Machine learning techniques have found numerous applications in trading stocks and forex. Some of the key areas where machine learning is being utilised include:
Pattern Recognition: Machine learning algorithms can identify complex patterns and relationships in historical market data. This can help traders identify recurring patterns, such as chart patterns or candlestick formations, that may indicate potential trading opportunities.
Predictive Modelling: By analysing historical data, machine learning models can make predictions about future market movements. These predictions can be used to inform trading decisions, such as when to buy or sell a particular asset.
Risk Management: Machine learning algorithms can assess and manage risk by analysing historical market data and identifying potential risks or anomalies. This can help traders optimise their risk-reward ratio and make more informed decisions about position sizing and stop-loss levels.
Algorithmic Trading: Machine learning techniques are widely used in developing algorithmic trading strategies. These strategies can automatically execute trades based on predefined rules and market conditions, eliminating human emotions and biases from the trading process.
Sentiment Analysis: Machine learning algorithms can analyse news articles, social media feeds, and other sources of information to gauge market sentiment. This can help traders understand the impact of news events on market movements and make more informed trading decisions.
Advantages of Machine Learning in Trading
The use of machine learning in trading stocks and forex offers several advantages:
Data-driven Decision Making: Machine learning algorithms can analyse vast amounts of data and extract meaningful insights. This enables traders to make more informed decisions based on data-driven analysis rather than relying solely on intuition or subjective judgement.
Improved Efficiency: Machine learning algorithms can process and analyse data much faster than humans. This allows traders to quickly identify trading opportunities, execute trades, and manage risk in real-time, leading to improved efficiency and potentially higher profits.
Ability to Handle Complex Data: Financial markets generate large volumes of complex data, including price data, news articles, economic indicators, and more. Machine learning algorithms can handle and analyse this data effectively, uncovering hidden patterns and relationships that may not be apparent to human traders.
Adaptability and Learning: Machine learning models can adapt and learn from new data, allowing them to continuously improve their performance over time. This adaptability is particularly valuable in dynamic and ever-changing financial markets.
Limitations of Machine Learning in Trading
While machine learning has shown great promise in trading stocks and forex, it also has some limitations:
Data Quality and Bias: Machine learning models heavily rely on the quality and representativeness of the data they are trained on. If the training data is biased or incomplete, the model's predictions may be inaccurate or biased as well.
Overfitting: Machine learning models can sometimes overfit the training data, meaning they become too specialised in capturing the noise or idiosyncrasies of the training data. This can lead to poor generalisation and inaccurate predictions based on new, unseen data.
Lack of Interpretability: Some machine learning models, such as deep learning neural networks, are often considered black boxes, making it challenging to interpret the reasoning behind their predictions. This lack of interpretability can be a concern in trading, where understanding the rationale behind a decision is crucial.
Market Complexity and Uncertainty: A wide range of factors, such as economic indicators, geopolitical events, and investor sentiment, have an impact on financial markets. Machine learning models may struggle to capture the full complexity and uncertainty of these markets, leading to suboptimal predictions or decisions.
Despite these limitations, machine learning continues to evolve and improve, and its application in trading stocks and forex is likely to grow in the future. Traders and investors who embrace these technologies and understand their strengths and limitations can gain a competitive edge in the ever-changing financial markets.
Deep learning in trading
Deep learning is a subset of machine learning that focuses on training artificial neural networks to learn from and make predictions from large amounts of data. It has gained significant attention in recent years due to its ability to handle complex patterns and relationships in data. In the context of trading stocks and forex, deep learning has emerged as a powerful tool for making predictions and generating trading signals.
Understanding deep learning
The structure and operation of the human brain serve as inspiration for deep learning. It involves the use of artificial neural networks, which are composed of interconnected layers of nodes called neurons. Each neuron takes inputs, applies a mathematical function to them, and produces an output. The outputs from one layer of neurons serve as inputs to the next layer, allowing the network to learn and extract higher-level features from the data.
One of the key advantages of deep learning is its ability to automatically learn and extract relevant features from raw data. This eliminates the need for manual feature engineering, which can be time-consuming and subjective. Deep learning models can learn complex patterns and relationships in the data, enabling them to make accurate predictions and generate trading signals.
Applications of Deep Learning in Trading
Deep learning has found numerous applications in trading stocks and forex. Some of the key areas where deep learning techniques have been successfully applied include:
1. Price prediction
Deep learning models can be trained to analyze historical price data and make predictions about future price movements. By learning from patterns and trends in the data, these models can generate trading signals indicating when to buy or sell a particular stock or currency pair. Deep learning models have shown promising results in predicting short-term price movements, which can be valuable for day traders and short-term investors.
2. Sentiment Analysis
Sentiment analysis involves analyzing news articles, social media posts, and other textual data to gauge market sentiment and its potential impact on stock and forex prices. Deep learning models can be trained to understand and interpret the sentiment expressed in text, allowing traders to make informed decisions based on market sentiment. By incorporating sentiment analysis into their trading strategies, traders can gain a deeper understanding of market dynamics and potentially identify profitable trading opportunities.
3. Pattern Recognition
Deep learning models excel at recognising complex patterns and relationships in data. In trading, this ability can be leveraged to identify recurring patterns in price charts, such as support and resistance levels, chart patterns, and trend reversals. By recognising these patterns, deep learning models can generate trading signals that help traders make more informed decisions. Pattern recognition using deep learning has the potential to improve the accuracy of technical analysis and enhance trading strategies.
4. Portfolio Optimisation
Deep learning can also be applied to optimise investment portfolios. By analysing historical data and considering various factors such as risk, return, and correlation, deep learning models can suggest optimal portfolio allocations. These models can adapt and learn from changing market conditions, allowing traders to adjust their portfolios accordingly. Portfolio optimisation using deep learning can help traders achieve better risk-adjusted returns and improve overall portfolio performance.
Advantages of Deep Learning in Trading
Deep learning offers several advantages in the context of trading stocks and forex:
1. Ability to Handle Complex Data
Deep learning models can handle large and complex datasets, including high-frequency trading data, news articles, and social media feeds. This allows traders to incorporate a wide range of information into their trading strategies, leading to more accurate predictions and better decision-making.
2. Automatic Feature Extraction
Deep learning models can automatically learn and extract relevant features from raw data. This eliminates the need for manual feature engineering, which can be time-consuming and subjective. By automatically learning features, deep learning models can capture complex patterns and relationships in the data, leading to more accurate predictions.
3. Adaptability and Flexibility
Deep learning models are highly adaptable and can learn from changing market conditions. They can continuously update their predictions and trading signals based on new data, allowing traders to adjust their strategies in real-time. This adaptability and flexibility make deep learning models well-suited for dynamic and fast-paced trading environments.
Limitations of Deep Learning in Trading
While deep learning has shown great promise in trading stocks and forex, it also has some limitations.
1. Data Requirements
Deep learning models typically require large amounts of data to train effectively. In trading, obtaining high-quality and relevant data can be challenging, especially for individual traders. Limited data availability can hinder the performance of deep learning models and lead to overfitting, where the model performs well on the training data but fails to generalise to new data.
2. Interpretability
Deep learning models are often considered black boxes, meaning that it can be challenging to understand and interpret the reasoning behind their predictions. This lack of interpretability can be a concern for traders who want to understand the underlying factors driving the model's decisions. However, efforts are being made to develop techniques that provide insights into the decision-making process of deep learning models.
3. Computational Resources
Training deep learning models can be computationally intensive and requires significant computational resources, including powerful hardware and large-scale computing infrastructure. This can be a barrier for individual traders or small firms with limited resources. However, advancements in cloud computing and the availability of pre-trained models have made deep learning more accessible to a wider range of traders.
Despite these limitations, deep learning continues to evolve and improve, and its applications in trading stocks and forex are likely to expand in the future. Traders who embrace deep learning techniques and leverage their advantages can gain a competitive edge in the market and potentially achieve better trading outcomes.
Natural language processing in trading
Natural Language Processing (NLP) is a branch of artificial intelligence that focuses on the interaction between computers and human language. It involves the ability of machines to understand, interpret, and generate human language in a way that is meaningful and useful. In the context of trading stocks and forex, NLP plays a crucial role in extracting valuable insights from textual data such as news articles, social media posts, and financial reports.
Understanding Natural Language Processing
NLP techniques enable computers to process and analyse large volumes of unstructured textual data, which is abundant in the financial markets. By applying NLP algorithms, traders and investors can gain valuable insights into market sentiment, news sentiment, and other relevant information that can impact stock and forex prices.
One of the key challenges in NLP is the ambiguity and complexity of human language. Words and phrases can have multiple meanings, and the context in which they are used can greatly influence their interpretation. NLP algorithms use various techniques, such as syntactic analysis, semantic analysis, and sentiment analysis, to overcome these challenges and extract meaningful information from textual data.
Applications of Natural Language Processing in Trading
Sentiment Analysis: NLP techniques can be used to analyse the sentiment expressed in news articles, social media posts, and other sources of information. By understanding the sentiment of market participants, traders can gauge the overall market sentiment and make informed trading decisions. For example, if there is a positive sentiment towards a particular stock or currency, it may indicate a potential buying opportunity.
News Analysis: NLP can be used to analyse news articles and extract key information such as company earnings, mergers and acquisitions, regulatory changes, and other events that can impact stock and forex prices. By automatically processing and analysing news articles, traders can stay updated with the latest market developments and adjust their trading strategies accordingly.
Event Detection: NLP algorithms can be used to detect and classify important events from textual data. For example, they can identify earnings announcements, central bank policy changes, and other significant events that can impact the financial markets. By automatically detecting these events, traders can react quickly and take advantage of market opportunities.
Textual Data Mining: NLP techniques can be used to mine valuable information from large volumes of textual data. For example, by analysing financial reports, earnings transcripts, and analyst reports, traders can identify patterns and trends that can help them make better trading decisions. NLP algorithms can also be used to extract key financial indicators such as revenue, earnings, and cash flow from textual data.
Benefits of Natural Language Processing in Trading
Automation and Efficiency: NLP algorithms can process and analyse large volumes of textual data much faster than humans. This enables traders to stay updated with the latest market information and make timely trading decisions. By automating the analysis of textual data, traders can save time and focus on other important aspects of trading.
Improved Decision Making: By extracting valuable insights from textual data, NLP techniques can help traders make more informed and data-driven trading decisions. For example, by analysing news sentiment, traders can identify potential market trends and adjust their trading strategies accordingly. NLP can also help traders identify trading opportunities based on the analysis of financial reports and other relevant information.
Market Sentiment Analysis: NLP algorithms can analyse the sentiment expressed in news articles, social media posts, and other sources of information. By understanding the overall market sentiment, traders can gauge the market's reaction to specific events and make informed trading decisions. For example, if there is a negative sentiment towards a particular stock, it may indicate a potential selling opportunity.
Event Detection and Reaction: NLP techniques can automatically detect and classify important events from textual data. By quickly identifying significant events, such as earnings announcements or regulatory changes, traders can react promptly and take advantage of market opportunities. This can help traders stay ahead of the competition and potentially generate higher returns.
Limitations of Natural Language Processing in Trading
Language Complexity: Human language is complex and often ambiguous. NLP algorithms may struggle to accurately interpret the meaning of certain words or phrases, especially in the context of financial markets. This can lead to the misinterpretation of textual data and potentially incorrect trading decisions.
Data Quality and Reliability: The accuracy and reliability of NLP analysis heavily depend on the quality of the underlying data. If the data used for analysis is incomplete, inaccurate, or biased, it can lead to misleading insights and unreliable trading decisions. Traders need to ensure that the data used for NLP analysis is of high quality and comes from reliable sources.
Lack of Contextual Understanding: NLP algorithms may struggle to understand the context in which certain words or phrases are used. This can lead to the misinterpretation of textual data and potentially incorrect trading decisions. Traders need to carefully consider the limitations of NLP algorithms and validate the insights generated through other means.
Market Noise and Manipulation: A number of factors, including market noise and manipulation, have an impact on the financial markets. NLP algorithms may struggle to differentiate between genuine market signals and noise generated by market manipulation or irrelevant information. Traders need to be cautious and use NLP insights in conjunction with other analysis techniques to make informed trading decisions.
Natural language processing (NLP) plays a significant role in trading stocks and forex by enabling the analysis of large volumes of textual data. NLP techniques such as sentiment analysis, news analysis, event detection, and textual data mining provide valuable insights that can help traders make informed trading decisions. However, it is important to be aware of the limitations of NLP, including language complexity, data quality and reliability, a lack of contextual understanding, and market noise and manipulation. Traders should use NLP insights as part of a comprehensive trading strategy and validate them through other analysis techniques.
Reinforcement learning in trading
Reinforcement learning (RL) is a branch of artificial intelligence (AI) that focuses on training agents to make decisions in an environment to maximize a reward signal. RL has gained significant attention in recent years due to its ability to learn complex strategies and adapt to dynamic environments. In the context of trading stocks and forex, RL has shown promise in developing intelligent trading systems that can make profitable decisions.
Understanding Reinforcement Learning
The way that people and animals learn through trial and error serves as an inspiration for reinforcement learning. The RL agent interacts with an environment and learns by receiving feedback in the form of rewards or penalties. The agent's goal is to learn the optimal policy that maximises the cumulative reward over time.
In the context of trading, the RL agent learns to make buy or sell decisions based on historical price data and other relevant market information. It takes action, observes the outcomes, and adjusts its strategy to maximise profits or minimise losses. RL algorithms use a combination of exploration and exploitation to strike a balance between trying out new actions and exploiting the known profitable ones.
Applications of Reinforcement Learning in Trading
Reinforcement learning has found various applications in trading stocks and forex. Some of the key areas where RL techniques have been successfully applied include:
1. Portfolio Management
RL can be used to optimise portfolio allocation by dynamically adjusting the weights of different assets based on market conditions. The RL agent learns to rebalance the portfolio to maximise returns while considering risk constraints. By continuously adapting to changing market conditions, RL-based portfolio management systems can potentially outperform traditional static allocation strategies.
2. Order Execution
RL algorithms can be used to optimise the execution of large orders by breaking them into smaller orders and determining the optimal timing and size of each trade. The RL agent learns to minimise market impact and transaction costs by considering factors such as liquidity, price volatility, and order book dynamics. This can lead to improved execution performance and reduced slippage.
3. Market making
Market making involves providing liquidity to the market by continuously quoting bid and ask prices. RL can be used to train market-making agents to dynamically adjust their quotes based on market conditions. The RL agent learns to balance the trade-off between profit and risk by considering factors such as order flow, volatility, and inventory management. RL-based market-making strategies have the potential to improve liquidity provision and reduce bid-ask spreads.
4. Trading Strategy Optimisation
RL can be used to optimise trading strategies by learning the optimal sequence of actions to maximise profits. The RL agent learns to adapt its strategy to different market regimes and exploit patterns in the data. By continuously learning and updating its policy, RL-based trading strategies can potentially adapt to changing market conditions and generate consistent profits.
Advantages of Reinforcement Learning in Trading
Reinforcement learning offers several advantages in the context of trading stocks and forex:
1. Adaptability
RL agents can adapt to changing market conditions and learn from experience. They can continuously update their strategies based on new data and adjust their decision-making process accordingly. This adaptability allows RL-based trading systems to stay relevant and profitable in dynamic and evolving markets.
2. Ability to Learn Complex Strategies
RL algorithms can learn complex strategies that may be difficult to define explicitly. They can discover patterns and exploit market inefficiencies that may not be apparent to human traders. RL-based trading systems have the potential to uncover hidden opportunities and generate superior returns compared to traditional trading approaches.
3. Handling Non-Stationary Environments
Financial markets are known for their non-stationary nature, where market dynamics change over time. RL algorithms are well-suited to handle such environments as they can adapt their strategies to changing market conditions. This flexibility allows RL-based trading systems to perform well even in highly volatile and unpredictable markets.
Challenges of Reinforcement Learning about trading
While reinforcement learning holds promise for trading stocks and forex, there are several challenges that need to be addressed:
1. Data Quality and Availability
Reinforcement learning algorithms require large amounts of high-quality data to learn effectively. Obtaining clean and reliable historical price data, as well as other relevant market information, can be a challenge. Additionally, the availability of real-time data for training RL agents in live trading environments can be limited.
2. Model Complexity and Interpretability
RL models can be complex and difficult to interpret. This lack of interpretability can make it challenging to understand the reasoning behind the decisions made by RL-based trading systems. It is crucial to strike a balance between model complexity and interpretability to ensure transparency and trust in the decision-making process.
3. Overfitting and generalisation
Overfitting is a common challenge in RL, where the agent becomes too specialised in the training data and fails to generalise well to new, unseen data. It is essential to design RL algorithms that can generalise their learned strategies to different market conditions and avoid overfitting to historical data.
4. Risk Management
RL-based trading systems need robust risk management mechanisms to handle potential losses. The inherent uncertainty and volatility of financial markets require careful consideration of risk factors. It is crucial to incorporate risk management techniques into RL algorithms to ensure the stability and long-term profitability of trading strategies.
Reinforcement learning has the potential to revolutionize the field of trading stocks and forex by developing intelligent and adaptive trading systems. RL-based approaches offer adaptability, the ability to learn complex strategies, and the capability to handle non-stationary environments. However, challenges related to data quality, model complexity, overfitting, and risk management need to be addressed to fully harness the power of RL in trading. With ongoing advancements in AI and the increasing availability of data, the future of reinforcement learning in trading looks promising.
Data Analysis and Prediction in Trading
Data collection and preprocessing are crucial steps in utilizing artificial intelligence (AI) in trading stocks and forex. In this section, we will explore the importance of data, the challenges in collecting and preprocessing it, and the techniques used to ensure high-quality data for AI-based trading systems.
Importance of Data in AI Trading
In AI trading, data is the foundation upon which predictive models and trading strategies are built. The quality and quantity of data directly impact the accuracy and reliability of AI algorithms. Therefore, it is essential to collect and preprocess data effectively to extract meaningful insights and make informed trading decisions.
Historical market data, such as price and volume information, is commonly used in AI trading. This data provides valuable insights into market trends, patterns, and correlations. Additionally, news articles, social media sentiment, economic indicators, and other relevant information can be incorporated to enhance the predictive power of AI models.
Challenges in Data Collection
Collecting data for AI trading poses several challenges. One of the primary challenges is the availability and accessibility of high-quality data. Market data can be expensive, especially for high-frequency trading, where real-time data is crucial. Moreover, obtaining accurate and reliable data from various sources can be time-consuming and complex.
Another challenge is the vast amount of data generated in financial markets. Handling and processing large datasets requires robust infrastructure and computational resources. Additionally, data from different sources may have inconsistencies, missing values, or errors, which need to be addressed during the preprocessing stage.
Data Preprocessing Techniques
Data preprocessing involves transforming raw data into a clean and structured format suitable for AI algorithms. Several techniques are employed to address the challenges mentioned above and ensure the quality of the data used in AI trading.
1. Data Cleaning: This step involves handling missing values, outliers, and inconsistencies in the data. Missing values can be imputed using various methods, such as mean imputation or interpolation. Outliers, which can significantly impact the performance of AI models, can be detected and treated using statistical techniques or domain knowledge.
2. Feature Selection and Extraction: In AI trading, not all features are equally relevant for predicting market movements. Feature selection techniques, such as correlation analysis or statistical tests, help identify the most informative features. Feature extraction methods, such as principal component analysis (PCA) or wavelet transforms, can be used to reduce the dimensionality of the data while preserving important information.
3. Normalisation and Scaling: Different features in the data may have different scales, which can affect the performance of AI algorithms. Normalization techniques, such as min-max scaling or z-score normalization, are applied to ensure that all features have a similar scale. This step helps prevent certain features from dominating the learning process.
4. Handling Time-Series Data: In trading, time-series data plays a crucial role. Techniques like resampling, smoothing, or lagging can be used to handle the temporal nature of the data. Additionally, creating lagged variables can capture the dependencies between past and future market movements.
5. Handling Imbalanced Data: In financial markets, imbalanced datasets are common, where the occurrence of one class (e.g., price increase) is significantly higher than the other (e.g., price decrease). Techniques like oversampling, undersampling, or synthetic minority oversampling technique (SMOTE) can be employed to address the class imbalance problem.
Data Quality and Bias
Ensuring data quality is essential to avoid biased or misleading results in AI trading. Biases can arise from various sources, such as data collection methods, sample selection, or human judgment. It is crucial to carefully evaluate the data sources, identify potential biases, and take appropriate measures to mitigate them.
Moreover, biases can also be introduced during the preprocessing stage. For example, if data cleaning or feature selection is performed without considering the underlying biases, it can lead to distorted results. Therefore, it is important to be aware of potential biases and regularly monitor and evaluate the performance of AI models to detect and correct any biases that may arise.
Data collection and preprocessing are fundamental steps in utilizing AI in trading stocks and forex. The availability of high-quality data and the application of appropriate preprocessing techniques are crucial for building accurate predictive models and effective trading strategies. By addressing the challenges in data collection and ensuring data quality, AI-based trading systems can leverage the power of AI to make informed and profitable trading decisions.
Technical analysis with AI
Technical analysis is a widely used approach in trading that involves analyzing historical price and volume data to make predictions about future market movements. Traditionally, technical analysis has been performed manually by traders, who rely on their expertise and intuition to identify patterns and trends in the data. However, with the advancements in artificial intelligence (AI), it is now possible to automate and enhance the technical analysis process.
AI techniques, such as machine learning and deep learning, have revolutionized the field of technical analysis by enabling computers to analyze vast amounts of data and identify complex patterns that may not be apparent to human traders. These AI-powered systems can process and analyze data at a much faster pace than humans, allowing for more efficient and accurate predictions.
One of the key advantages of using AI in technical analysis is its ability to identify patterns and trends that may not be easily detectable by human traders. AI algorithms can analyze large datasets and identify subtle correlations and patterns that may be indicative of future market movements. This can help traders make more informed decisions and potentially increase their profitability.
Another benefit of using AI in technical analysis is its ability to remove human biases and emotions from the trading process. Human traders frequently succumb to emotions like fear and greed, which can impair their judgment and result in irrational decision-making. On the other hand, AI systems are immune to emotion and are capable of making fact-based predictions using historical data.
AI-powered technical analysis systems can also adapt and learn from new data, allowing them to continuously improve their predictions over time. These systems can analyze real-time market data and adjust their models and strategies accordingly, ensuring that they remain up-to-date and effective in dynamic market conditions.
However, it is important to acknowledge the limitations and challenges associated with using AI in technical analysis. One of the main challenges is the availability and quality of data. AI algorithms rely on historical data to make predictions, and if the data is incomplete or inaccurate, it can lead to unreliable predictions. Therefore, it is crucial to ensure that the data used for training AI models is of high quality and representative of market conditions.
Another challenge is the interpretability of AI models. While AI algorithms can generate accurate predictions, they often lack transparency in explaining how they arrived at those predictions. This lack of interpretability can make it difficult for traders to trust and understand the decisions made by AI systems. Addressing this challenge is crucial to gaining wider acceptance and adoption of AI in technical analysis.
Furthermore, AI-powered technical analysis systems are not immune to market risks and uncertainties. While they can analyze historical data and identify patterns, they cannot predict unforeseen events or sudden market shifts. Traders must still exercise caution and consider other factors, such as economic indicators and geopolitical events when making trading decisions.
AI has the potential to revolutionise technical analysis in trading stocks and forex. By leveraging AI techniques, traders can benefit from more accurate predictions, faster analysis, and reduced human biases. However, it is important to recognise the challenges associated with using AI, such as data quality, interpretability, and the limitations of relying solely on historical data. Traders should view AI as a powerful tool that complements their decision-making process rather than a replacement for human judgment. With proper understanding and utilisation, AI can significantly enhance the effectiveness and efficiency of technical analysis in trading.
Fundamental Analysis with AI
Fundamental analysis is a crucial aspect of trading in both the stock and forex markets. It involves evaluating the intrinsic value of an asset by analysing various economic, financial, and qualitative factors that can influence its price. Traditionally, fundamental analysis has been a manual and time-consuming process, requiring traders to gather and analyse vast amounts of data. However, with the advent of artificial intelligence (AI), fundamental analysis has been revolutionised, making it more efficient and accurate.
AI has the potential to enhance fundamental analysis by automating data collection, processing, and interpretation. By leveraging AI techniques such as machine learning, natural language processing, and predictive modelling, traders can gain valuable insights into the financial health and future prospects of companies and economies. This section explores the application of AI in fundamental analysis and its impact on trading in both stocks and forex markets.
Automating Data Collection and Preprocessing
One of the primary challenges in fundamental analysis is the collection and preprocessing of vast amounts of data from various sources. AI can automate this process by utilizing web scraping techniques to gather data from financial statements, news articles, social media, and other relevant sources. Natural language processing algorithms can then be applied to extract key information and sentiments from textual data, enabling traders to analyse the impact of news and events on asset prices.
Enhancing Financial Statement Analysis
Financial statements provide valuable insights into a company's financial health and performance. AI can assist in analysing these statements by automatically extracting and interpreting relevant information. Machine learning algorithms can identify patterns and trends in financial data, enabling traders to assess a company's profitability, liquidity, solvency, and growth potential. By automating financial statement analysis, AI can save time and reduce human errors, allowing traders to make more informed investment decisions.
Analysing Economic Indicators and News
Economic indicators and news play a significant role in fundamental analysis. AI can analyse vast amounts of economic data, such as GDP growth, inflation rates, interest rates, and employment figures, to assess the overall health of an economy. By incorporating these indicators into predictive models, AI can forecast the impact of economic events on asset prices. Additionally, AI can analyse news articles and social media sentiment to gauge market sentiment and identify potential market-moving events.
Predictive modeling for valuation
Valuing assets accurately is crucial for successful trading. AI can assist in predicting asset valuations by developing sophisticated predictive models. Machine learning algorithms can analyse historical data and identify patterns and relationships between various factors and asset prices. By incorporating these models into fundamental analysis, traders can estimate the fair value of assets and identify potential opportunities for buying or selling.
Risk Assessment and Management
AI can also play a vital role in risk assessment and management through fundamental analysis. By analysing historical data and market trends, AI algorithms can identify potential risks and assess their impact on asset prices. This information can help traders develop risk management strategies, such as setting stop-loss orders or diversifying their portfolios. Additionally, AI can continuously monitor market conditions and alert traders to potential risks or opportunities in real time.
Limitations and Challenges
While AI offers significant advantages in fundamental analysis, it is essential to acknowledge its limitations and challenges. AI models heavily rely on historical data, and their accuracy may be compromised during unprecedented events or market disruptions. Additionally, AI models can be sensitive to data quality and biases, which can affect the accuracy of their predictions. Traders must also be cautious of over-reliance on AI and ensure that human judgment and expertise are still incorporated into the decision-making process.
AI has the potential to revolutionize fundamental analysis in trading stocks and forex. By automating data collection, enhancing financial statement analysis, analyzing economic indicators and news, developing predictive models, and assisting in risk assessment and management, AI can provide traders with valuable insights and improve their decision-making process. However, it is crucial to understand the limitations and challenges associated with AI and ensure that it is used as a tool to augment human expertise rather than replace it. As AI continues to evolve, its impact on fundamental analysis and trading is expected to grow, shaping the future of the financial markets.
Predictive modeling in trading
Predictive modeling is a powerful technique in trading that utilizes artificial intelligence (AI) to forecast future market movements and make informed trading decisions. By analysing historical data and identifying patterns, predictive models can provide traders with valuable insights into potential market trends and help them anticipate price movements. In this section, we will explore the concept of predictive modeling in trading and discuss its benefits and limitations.
Understanding predictive modelling
Predictive modeling involves the use of statistical algorithms and machine learning techniques to create models that can predict future outcomes based on historical data. In the context of trading, predictive models are trained on historical market data, such as price and volume, to identify patterns and relationships that can be used to forecast future market movements.
The process of building a predictive model typically involves several steps. First, the trader needs to collect and preprocess the relevant data, ensuring that it is clean and suitable for analysis. Next, the trader selects an appropriate algorithm or machine learning technique to train the model. The model is then trained on the historical data, and its performance is evaluated using various metrics. Once the model is deemed accurate and reliable, it can be used to make predictions on new, unseen data.
Benefits of Predictive Modelling in Trading
Predictive modeling offers several benefits to traders in the stock and forex markets. Here are some of the key advantages:
Improved Decision Making: By leveraging predictive models, traders can make more informed decisions based on data-driven insights. These models can identify patterns and trends that may not be apparent to human traders, enabling them to make more accurate predictions about future market movements.
Enhanced Risk Management: Predictive models can help traders assess and manage risk more effectively. By analysing historical data and identifying potential risks, traders can adjust their trading strategies and take appropriate measures to mitigate potential losses.
Increased Efficiency: Predictive modelling automates the process of analysing large volumes of data, saving traders time and effort. By using AI algorithms to analyse data and generate predictions, traders can focus on other aspects of their trading strategies, such as portfolio management and execution.
Opportunity Identification: Predictive models can identify potential trading opportunities that may not be obvious to human traders. By analysing historical data and identifying patterns, these models can uncover hidden trends and anomalies that can be exploited for profit.
Quantitative Analysis: Predictive modelling provides traders with a quantitative approach to trading. By relying on data and statistical analysis, traders can make decisions based on objective criteria rather than subjective judgement, reducing the impact of emotions on trading outcomes.
Limitations of Predictive Modelling in Trading
While predictive modelling offers significant benefits, it is important to acknowledge its limitations. Here are some of the key challenges associated with predictive modelling in trading:
Data Quality and Availability: The accuracy and reliability of predictive models depend on the quality and availability of data. If the historical data used to train the model is incomplete, inaccurate, or biased, the predictions generated by the model may be unreliable.
Changing Market Conditions: Predictive models are built on historical data, which may not accurately reflect current market conditions. Market dynamics can change rapidly, rendering historical patterns and relationships less relevant. Traders need to continuously update and adapt their models to account for changing market conditions.
Overfitting and Generalisation: Predictive models can be prone to overfitting, where they perform well on historical data but fail to generalise to new, unseen data. Traders need to carefully validate and evaluate their models to ensure that they are not overfitting to noise or random patterns in the data.
Model Complexity and Interpretability: Some predictive models, such as deep learning models, can be highly complex and difficult to interpret. This lack of interpretability can make it challenging for traders to understand and trust the predictions generated by these models.
Black Box Nature: Predictive models can sometimes be seen as black boxes, where the inner workings and decision-making processes are not transparent. This lack of transparency can raise concerns about the fairness and accountability of AI-driven trading systems.
Despite these limitations, predictive modelling remains a valuable tool for traders in the stock and forex markets. By leveraging AI and machine learning techniques, traders can gain valuable insights into market trends and make more informed trading decisions. However, it is important for traders to understand the limitations of predictive modelling and use it as part of a broader trading strategy that incorporates other forms of analysis and risk management techniques.
Risk Assessment and Management with AI
Risk assessment and management are crucial aspects of trading in both stocks and forex markets. The use of artificial intelligence (AI) can greatly enhance these processes by providing traders with advanced tools and techniques to identify, measure, and mitigate risks. In this section, we will explore how AI can be utilised for risk assessment and management in trading, along with its benefits and limitations.
Understanding Risk Assessment
Before delving into the role of AI in risk assessment and management, it is important to understand the concept of risk assessment itself. Risk assessment involves the identification and evaluation of potential risks associated with trading activities. These risks can include market volatility, economic factors, geopolitical events, and other factors that may impact the performance of stocks or forex pairs.
Traditionally, risk assessment in trading has relied on manual analysis and subjective judgement. Traders would analyse historical data, market trends, and news events to assess the potential risks involved in a particular trade. However, this approach is time-consuming, prone to human biases, and may not fully capture the complexity of the trading environment.
The role of AI in risk assessment
AI can play a significant role in risk assessment by leveraging its ability to process vast amounts of data, identify patterns, and make data-driven predictions. By utilising AI algorithms, traders can automate the risk assessment process and gain valuable insights into potential risks and their impact on trading strategies.
One of the key advantages of AI in risk assessment is its ability to analyse both structured and unstructured data. Structured data includes historical price data, financial statements, and economic indicators, while unstructured data encompasses news articles, social media sentiment, and other textual data. By analysing this diverse range of data sources, AI algorithms can identify hidden patterns and correlations that may not be apparent to human traders.
AI can also assist in real-time risk assessment by continuously monitoring market conditions and adjusting trading strategies accordingly. By analysing market data in real-time, AI algorithms can identify emerging risks and adapt trading strategies to minimise potential losses. This dynamic risk assessment capability can be particularly valuable in fast-paced trading environments, where market conditions can change rapidly.
Benefits of AI in Risk Assessment and Management
The integration of AI into risk assessment and management processes offers several benefits for traders:
1. Enhanced Accuracy: AI algorithms can process vast amounts of data with speed and accuracy, reducing the likelihood of human errors in risk assessment. This can lead to more reliable risk predictions and better-informed trading decisions.
2. Improved Efficiency: AI can automate the risk assessment process, saving traders significant time and effort. By leveraging AI tools, traders can analyse large datasets and generate risk reports in a fraction of the time it would take manually.
3. Advanced Analytics: AI algorithms can perform complex data analysis and identify patterns that may not be apparent to human traders. This can provide traders with valuable insights into market trends, correlations, and potential risks.
4. Real-time Monitoring: AI can continuously monitor market conditions and adjust trading strategies in real-time. This enables traders to respond quickly to changing market dynamics and mitigate potential risks.
5. Risk Mitigation: By accurately identifying potential risks, AI can help traders develop effective risk mitigation strategies. This can involve adjusting position sizes, setting stop-loss orders, or diversifying portfolios to minimise potential losses.
Limitations of AI in Risk Assessment and Management
While AI offers significant benefits in risk assessment and management, it is important to acknowledge its limitations.
1. Data Quality: The accuracy and reliability of AI predictions heavily depend on the quality of the data used for training. If the training data is incomplete, biased, or outdated, it can lead to inaccurate risk assessments and flawed trading decisions.
2. Overreliance on Historical Data: AI algorithms primarily rely on historical data to make predictions. However, financial markets are dynamic and subject to changing conditions. If market conditions deviate significantly from historical patterns, AI models may struggle to accurately assess risks.
3. Lack of Contextual Understanding: While AI algorithms can analyse vast amounts of data, they may lack the contextual understanding that human traders possess. This can limit their ability to interpret complex events, such as geopolitical developments or unexpected market shocks.
4. Black Box Nature: Some AI algorithms, such as deep learning models, operate as black boxes, making it challenging to understand the reasoning behind their predictions. This lack of transparency can raise concerns regarding the reliability and accountability of AI-driven risk assessments.
AI has the potential to revolutionise risk assessment and management in trading by providing traders with advanced tools and techniques to identify, measure, and mitigate risks. By leveraging AI algorithms, traders can enhance the accuracy and efficiency of risk assessments, gain valuable insights into potential risks, and develop effective risk mitigation strategies. However, it is important to recognise the limitations of AI in risk assessment, including data quality issues, overreliance on historical data, a lack of contextual understanding, and the black box nature of some AI models. Traders should carefully consider these factors and combine AI-driven risk assessments with human judgement to make well-informed trading decisions.
Backtesting and performance evaluation
Backtesting and performance evaluation are crucial steps in the development and implementation of trading strategies. These processes allow traders to assess the effectiveness and profitability of their strategies before deploying them in real-time trading. With the advent of artificial intelligence (AI) in trading, backtesting and performance evaluation have become even more sophisticated and accurate.
Backtesting
Backtesting refers to the process of evaluating a trading strategy using historical data to simulate how it would have performed in the past. It involves applying the strategy's rules to historical market data and analysing the resulting trades and performance metrics. AI techniques have greatly enhanced the capabilities of backtesting by enabling traders to analyse vast amounts of data and identify patterns and trends that may not be apparent to human traders.
AI-powered backtesting systems can process large datasets quickly and efficiently, allowing traders to test their strategies on a wide range of market conditions. These systems can also incorporate complex algorithms and machine learning models to optimise trading strategies based on historical data. By backtesting their strategies, traders can gain valuable insights into the strengths and weaknesses of their approaches and make informed decisions about their trading strategies.
Performance Evaluation
Performance evaluation is the process of assessing the profitability and risk associated with a trading strategy. It involves analysing various performance metrics, such as returns, risk-adjusted returns, drawdowns, and the Sharpe ratio, to gauge the effectiveness of the strategy. AI techniques can significantly enhance performance evaluation by providing more accurate and comprehensive assessments of trading strategies.
AI-powered performance evaluation systems can analyse large amounts of data and generate detailed performance reports in real-time. These systems can also incorporate advanced machine learning algorithms to identify patterns and trends in trading performance and provide actionable insights for traders. By leveraging AI in performance evaluation, traders can make data-driven decisions and continuously improve their trading strategies.
Advantages of AI in Backtesting and Performance Evaluation
The integration of AI in backtesting and performance evaluation offers several advantages for traders:
Efficiency: AI-powered systems can process large datasets quickly and efficiently, allowing traders to analyse a vast amount of historical data in a short period of time. This enables traders to test their strategies on a wide range of market conditions and make informed decisions based on comprehensive data analysis.
Accuracy: AI techniques can identify patterns and trends in historical data that may not be apparent to human traders. By leveraging machine learning algorithms, AI-powered systems can provide more accurate and comprehensive assessments of trading strategies, enabling traders to make data-driven decisions.
Automation: AI-powered backtesting and performance evaluation systems can automate the process of analysing historical data and generating performance reports. This saves traders significant time and effort, allowing them to focus on developing and refining their trading strategies.
Optimisation: AI techniques can optimise trading strategies based on historical data by incorporating complex algorithms and machine learning models. This enables traders to identify the most profitable and risk-efficient strategies and continuously improve their performance over time.
Limitations of AI in Backtesting and Performance Evaluation
While AI offers significant advantages in backtesting and performance evaluation, there are also some limitations to consider:
Data Quality: The accuracy and reliability of backtesting and performance evaluation heavily depend on the quality of the historical data used. AI-powered systems may produce inaccurate results if the historical data contains errors, gaps, or biases. Traders need to ensure the quality and integrity of the data used for backtesting and performance evaluation.
Overfitting: AI techniques can sometimes lead to overfitting, where a trading strategy performs exceptionally well on historical data but fails to generalise to new market conditions. Traders need to be cautious about over-optimising their strategies based on historical data and ensure that their strategies are robust and adaptable to changing market conditions.
Lack of Contextual Understanding: AI-powered systems may lack the contextual understanding and intuition that human traders possess. While AI can analyse vast amounts of data and identify patterns, it may struggle to interpret the underlying economic, geopolitical, or market factors that influence trading performance. Traders need to complement AI analysis with their domain expertise and market knowledge.
Model Risks: AI models used in backtesting and performance evaluation are not immune to risks. Models can be sensitive to changes in market conditions or assumptions made during the development process. Traders need to regularly monitor and update their AI models to ensure their accuracy and reliability.
AI has revolutionised backtesting and performance evaluation in trading by enabling traders to analyse vast amounts of data, identify patterns, and optimise their strategies. AI-powered systems offer efficiency, accuracy, automation, and optimisation capabilities that can significantly enhance traders' decision-making processes. However, traders need to be aware of the limitations of AI, such as data quality issues, overfitting, a lack of contextual understanding, and model risks. By leveraging AI while considering these limitations, traders can improve their trading strategies and make more informed decisions in the dynamic world of stocks and forex trading.
Algorithmic Trading with AI
Introduction to Algorithmic Trading
Algorithmic trading, also known as automated trading or black-box trading, is a trading strategy that utilises computer algorithms to execute trades in financial markets. With the advancement of technology and the rise of artificial intelligence (AI), algorithmic trading has become increasingly popular in recent years. This section will provide an introduction to algorithmic trading and its benefits in the context of AI.
What is algorithmic trading?
Algorithmic trading involves the use of pre-programmed instructions to automatically execute trades based on a set of predefined rules and conditions. These rules are typically based on various technical indicators, market trends, and statistical models. By leveraging AI techniques, algorithmic trading systems can analyse vast amounts of data, identify patterns, and make trading decisions in real-time.
The Role of AI in Algorithmic Trading
AI plays a crucial role in algorithmic trading by enabling the development of sophisticated trading strategies and enhancing decision-making processes. AI algorithms can process and analyse large volumes of data, including historical price data, news articles, social media sentiment, and economic indicators, to identify trading opportunities and make predictions about future market movements.
One of the key advantages of AI in algorithmic trading is its ability to adapt and learn from new information. Machine learning algorithms can continuously analyse market data and adjust trading strategies accordingly, improving their performance over time. This adaptive nature of AI allows algorithmic trading systems to quickly respond to changing market conditions and exploit profitable opportunities.
Benefits of Algorithmic Trading with AI
Algorithmic trading with AI offers several benefits for traders and investors:
Speed and Efficiency: AI-powered algorithms can execute trades at high speeds, enabling traders to take advantage of short-term market fluctuations and capitalise on price discrepancies. This speed and efficiency can lead to improved trade execution and reduced transaction costs.
Elimination of Emotional Bias: Emotions can often cloud judgement and lead to irrational trading decisions. On the other hand, AI algorithms can make unbiased trading decisions using data and predetermined rules because they are not subject to emotional influence. This eliminates the potential for emotional bias and improves overall trading performance.
Ability to Process Large Amounts of Data: Financial markets generate vast amounts of data every second. AI algorithms can efficiently process and analyse this data to identify patterns and trends that may not be apparent to human traders. This ability to handle big data allows algorithmic trading systems to make more informed and data-driven trading decisions.
24/7 Market Monitoring: AI-powered algorithmic trading systems can continuously monitor the market and execute trades round the clock, even when human traders are not available. This ensures that trading opportunities are not missed and allows for greater market coverage.
Risk Management: AI algorithms can incorporate risk management techniques into trading strategies, such as stop-loss orders and position sizing, to mitigate potential losses. By automatically adjusting risk parameters based on market conditions, algorithmic trading systems can help traders manage risk more effectively.
Limitations of Algorithmic Trading with AI
While algorithmic trading with AI offers numerous benefits, it also has some limitations that traders should be aware of:
Dependency on Historical Data: AI algorithms rely heavily on historical data to make predictions and identify patterns. However, past performance may not always be indicative of future results, and market conditions can change rapidly. Traders should be cautious of overfitting models to historical data and ensure that their algorithms are adaptable to changing market dynamics.
Technical Complexity: Developing and implementing AI-powered algorithmic trading systems requires technical expertise and resources. Traders need to have a solid understanding of AI techniques, programming languages, and data analysis to effectively build and maintain these systems. Additionally, the infrastructure required to support algorithmic trading can be costly and complex.
System Failures and Risks: Algorithmic trading systems are susceptible to technical glitches, connectivity issues, and data inaccuracies, which can lead to significant financial losses. Traders should have robust risk management protocols in place and regularly monitor and test their systems to minimise the risk of system failures.
Regulatory and Ethical Considerations: The use of AI in algorithmic trading raises regulatory and ethical concerns. Traders need to ensure that their algorithms comply with relevant regulations and do not engage in illegal or unethical trading practices. Additionally, the use of AI algorithms can introduce biases and unfairness in trading decisions, which need to be carefully addressed.
Despite these limitations, algorithmic trading with AI has the potential to revolutionise the financial markets by improving trading efficiency, reducing human error, and uncovering new trading opportunities. As technology continues to advance and AI techniques evolve, the future of algorithmic trading looks promising. Traders who embrace AI-powered algorithms and adapt to the changing landscape of the financial markets are likely to gain a competitive edge in the years to come.
Building trading strategies with AI
Artificial intelligence (AI) has revolutionised the way trading strategies are developed and implemented in the financial markets. With the ability to analyse vast amounts of data and make complex decisions in real-time, AI has become an indispensable tool for traders looking to gain a competitive edge. In this section, we will explore the process of building trading strategies with AI and discuss the benefits and challenges associated with this approach.
Understanding AI in trading
Before delving into the specifics of building trading strategies with AI, it is important to have a clear understanding of what AI entails in the context of trading. AI refers to the development of computer systems that can perform tasks that would typically require human intelligence. In the case of trading, AI algorithms are designed to analyse market data, identify patterns, and make trading decisions based on predefined rules or learned patterns.
The Role of AI in Building Trading Strategies
AI plays a crucial role in building trading strategies by leveraging its ability to process and analyse vast amounts of data. Traditional trading strategies often rely on technical indicators or fundamental analysis, which can be time-consuming and subjective. AI, on the other hand, can quickly analyse historical price data, news articles, social media sentiment, and other relevant information to identify patterns and generate trading signals.
One of the key advantages of using AI in building trading strategies is its ability to adapt to and learn from changing market conditions. AI algorithms can continuously analyse market data and adjust their trading rules accordingly, allowing traders to stay ahead of the curve and capitalise on emerging opportunities.
Benefits of Building Trading Strategies with AI
There are several benefits associated with building trading strategies with AI:
Data-driven Decision Making: AI algorithms can process vast amounts of data and identify patterns that may not be apparent to human traders. This data-driven approach can lead to more informed and objective trading decisions.
Speed and Efficiency: AI algorithms can analyse market data and generate trading signals in real-time, enabling traders to execute trades quickly and efficiently. This speed advantage is particularly crucial in high-frequency trading, where even a fraction of a second can make a significant difference.
Improved Accuracy: AI algorithms can analyse historical data and identify patterns that have led to successful trades in the past. By leveraging this historical knowledge, AI can potentially improve the accuracy of trading strategies and increase the likelihood of profitable trades.
Reduced Emotional Bias: Human traders frequently succumb to emotions like fear and greed, which can result in irrational trading decisions. AI algorithms, on the other hand, are not subject to emotional biases and can make objective decisions based on predefined rules or learned patterns.
Backtesting and Optimisation: AI algorithms can be backtested using historical data to evaluate their performance and optimise their parameters. This allows traders to fine-tune their strategies and identify the most profitable approach before deploying them in live trading.
Challenges of Building Trading Strategies with AI
While there are numerous benefits to using AI in building trading strategies, there are also several challenges that traders need to be aware of:
Data Quality and Availability: AI algorithms heavily rely on high-quality and relevant data to make accurate predictions. However, obtaining such data can be challenging, especially for individual traders who may not have access to comprehensive market data or proprietary datasets.
Overfitting and Generalisation: AI algorithms can sometimes overfit historical data, meaning they become too specialised in capturing past patterns but fail to generalise well to new market conditions. Traders need to carefully validate their AI models to ensure they can perform well in real-time trading.
Complexity and Interpretability: AI algorithms, particularly deep learning models, can be highly complex and difficult to interpret. This lack of interpretability can make it challenging for traders to understand the reasoning behind the AI's trading decisions, potentially leading to a lack of trust in the system.
Risk Management: While AI algorithms can generate profitable trading signals, they are not foolproof and can still result in losses. Traders need to implement robust risk management strategies to mitigate the potential risks associated with AI-based trading strategies.
Building trading strategies with AI offers numerous benefits, including data-driven decision-making, speed and efficiency, improved accuracy, reduced emotional bias, and the ability to backtest and optimise strategies. However, traders must also be aware of the challenges associated with AI, such as data quality and availability, overfitting, complexity, and risk management. By understanding these pros and cons, traders can effectively harness the power of AI to develop successful trading strategies and navigate the ever-evolving financial markets.
Automated Execution and Order Management
Automated execution and order management are crucial components of algorithmic trading systems that leverage artificial intelligence (AI) techniques. These systems aim to execute trades efficiently and effectively, taking advantage of market opportunities in real-time. By automating the execution process, traders can eliminate human error and emotions and achieve faster and more accurate trade execution.
Benefits of Automated Execution
Automated execution offers several benefits to traders in the stock and forex markets. Here are some of the key advantages:
Speed and Efficiency: AI-powered algorithms can execute trades at lightning-fast speeds, enabling traders to take advantage of market opportunities as soon as they arise. Automated systems can process vast amounts of data and execute trades in milliseconds, ensuring that traders can capitalise on even the smallest price movements.
Elimination of Human Error: Human traders are prone to making mistakes, whether due to fatigue, emotions, or cognitive biases. Automated execution eliminates these errors by following pre-defined rules and executing trades based on objective criteria. This reduces the risk of costly errors and improves overall trading performance.
Consistency: Automated execution ensures that trades are executed consistently according to predefined strategies. This eliminates the impact of human emotions and biases, which can lead to inconsistent decision-making. By maintaining consistency, traders can have greater confidence in their trading strategies and improve their long-term performance.
24/7 Trading: Unlike human traders who need rest, automated systems can operate 24/7, taking advantage of trading opportunities across different time zones. This allows traders to capture opportunities in global markets and react to news and events that may impact prices outside regular trading hours.
Risk Management: Automated execution systems can incorporate risk management techniques to protect traders from excessive losses. These systems can automatically set stop-loss orders, trailing stops, and other risk management parameters to limit potential losses and protect profits. By enforcing risk management rules consistently, traders can mitigate the impact of adverse market conditions.
Order management with AI
Order management is a critical aspect of automated trading systems. AI techniques can enhance order management by optimising trade execution and minimising market impact. Here are some ways AI is used in order management:
Smart Order Routing: AI algorithms can analyse market conditions, liquidity, and order book data to determine the optimal execution venue for a trade. By considering factors such as price, volume, and speed of execution, smart order routing algorithms can minimise slippage and improve trade execution quality.
Market Impact Analysis: AI models can analyse historical data and market conditions to estimate the potential impact of a large order on market prices. By predicting market impact, traders can adjust their trading strategies and execution algorithms to minimise price impact and avoid excessive market movements.
Order Slicing: AI algorithms can slice large orders into smaller, more manageable chunks to execute them gradually over time. This approach reduces the impact of large orders on market prices and helps traders achieve better average execution prices.
Order Prioritisation: AI algorithms can prioritise orders based on various factors such as urgency, profitability, and market conditions. By dynamically adjusting the order priority, traders can ensure that the most important trades are executed promptly, while less critical trades can be delayed or cancelled if market conditions change.
Challenges of Automated Execution
While automated execution offers numerous benefits, there are also challenges that traders need to consider:
Technical Complexity: Implementing automated execution systems requires advanced technical expertise and infrastructure. Traders need to develop or acquire AI algorithms, establish connectivity with trading platforms, and ensure robust data feeds. Additionally, maintaining and monitoring these systems requires ongoing technical support.
Data Quality and Reliability: AI algorithms heavily rely on data for decision-making. Traders need access to high-quality and reliable data to ensure accurate trade execution. Any issues with data quality, such as missing or incorrect data, can lead to suboptimal execution and potentially significant losses.
Over-Optimisation: Traders must be cautious about over-optimising their AI algorithms. Over-optimisation occurs when algorithms are fine-tuned to perform exceptionally well on historical data but fail to generalise to new market conditions. This can lead to poor performance in live trading and unexpected losses.
Regulatory Compliance: Automated execution systems must comply with regulatory requirements, such as order handling rules, market manipulation prevention, and best execution practices. Traders need to ensure that their AI algorithms and order management processes adhere to these regulations to avoid legal and financial consequences.
Automated execution and order management are integral parts of AI-powered trading systems. By leveraging AI techniques, traders can achieve faster and more efficient trade execution, eliminate human errors, and improve overall trading performance. However, traders must also be aware of the challenges associated with implementing and maintaining these systems, such as technical complexity, data quality, over-optimisation, and regulatory compliance. By understanding these challenges and leveraging the benefits of automated execution, traders can harness the power of AI to enhance their trading strategies and stay competitive in the dynamic world of stocks and forex trading.
High-frequency trading with AI
High-frequency trading (HFT) is a trading strategy that relies on the use of powerful computers and algorithms to execute a large number of trades in a fraction of a second. This strategy aims to take advantage of small price discrepancies and market inefficiencies that may exist for only a brief period of time. With the advent of artificial intelligence (AI), HFT has become even more sophisticated and prevalent in the financial markets.
Understanding High-Frequency Trading
High-frequency trading involves the use of complex algorithms that analyse vast amounts of market data and execute trades at lightning-fast speeds. These algorithms are designed to identify patterns, trends, and anomalies in the market and make split-second decisions to buy or sell securities. The goal is to capitalise on these opportunities and generate profits from small price movements.
AI has revolutionised high-frequency trading by enabling algorithms to learn from historical data and adapt to changing market conditions. Machine learning algorithms can analyse large datasets and identify patterns that may not be apparent to human traders. This allows HFT algorithms to make more accurate predictions and execute trades with greater precision.
Benefits of High-Frequency Trading with AI
Speed and Efficiency: AI-powered HFT algorithms can execute trades in a matter of microseconds, far faster than any human trader could. This speed allows HFT firms to take advantage of fleeting market opportunities and react to market changes in real-time.
Increased Liquidity: HFT algorithms provide liquidity to the market by constantly buying and selling securities. This helps to narrow bid-ask spreads and ensures that there is always a buyer or seller available for a trade. Increased liquidity benefits all market participants by reducing transaction costs and improving market efficiency.
Improved Market Quality: HFT algorithms help to eliminate market inefficiencies by quickly identifying and exploiting price discrepancies. This leads to more accurate pricing and reduces the impact of market manipulation. As a result, the overall quality of the market improves, benefiting all participants.
Enhanced Risk Management: AI-powered HFT algorithms can analyse vast amounts of data and identify potential risks in real-time. This allows traders to implement risk management strategies more effectively and reduces the likelihood of significant losses. HFT algorithms can also react quickly to changing market conditions and adjust trading strategies accordingly.
Increased Market Transparency: HFT algorithms generate a large amount of data, which can be analysed to gain insights into market behaviour. This data can help regulators and market participants better understand market dynamics and detect any potential market abuses or manipulations.
Limitations and Challenges of High-Frequency Trading with AI
Market Volatility: HFT algorithms are designed to take advantage of small price movements, but they can also exacerbate market volatility. In times of extreme market stress, HFT algorithms may amplify price swings and contribute to market instability.
Technical Risks: HFT relies heavily on technology infrastructure and connectivity. Any technical glitch or failure can disrupt trading operations and lead to significant losses. HFT firms need to invest heavily in robust and redundant systems to mitigate these risks.
Regulatory Concerns: The rapid and automated nature of HFT raises regulatory concerns. Regulators need to ensure that HFT firms operate in a fair and transparent manner and do not engage in manipulative or predatory trading practices. There is an ongoing debate about the need for stricter regulations to prevent market abuse.
Data Quality and Bias: AI algorithms rely on historical data to make predictions and decisions. If the data used is biased or of poor quality, it can lead to inaccurate predictions and suboptimal trading decisions. Ensuring the quality and integrity of data is crucial for the success of AI-powered HFT.
Ethical Considerations: HFT algorithms can execute a large number of trades within a short period, potentially impacting market stability and fairness. There are ethical concerns about the impact of HFT on market integrity and whether it gives an unfair advantage to certain market participants.
High-frequency trading with AI has transformed the financial markets, providing increased liquidity, improved market quality, and enhanced risk management. The speed and efficiency of AI-powered HFT algorithms allow traders to capitalise on small price movements and react to market changes in real-time. However, there are also limitations and challenges associated with HFT, including market volatility, technical risks, regulatory concerns, data quality, and ethical considerations. As AI continues to advance, it is essential to strike a balance between the benefits and risks of high-frequency trading to ensure fair and efficient markets in the future.
AI in Forex Trading
Overview of the Forex Market
The foreign exchange market, commonly known as the forex market, is the largest and most liquid financial market in the world. It operates 24 hours a day, five days a week, and facilitates the exchange of currencies between participants, including banks, corporations, governments, and individual traders. The forex market plays a crucial role in international trade and investment, as it allows for the conversion of one currency into another.
Size and Liquidity
The forex market boasts an impressive daily trading volume, with estimates ranging from $5 trillion to $6.6 trillion. This immense size is a result of the global nature of the market, as currencies from all over the world are traded. The high liquidity of the forex market ensures that traders can enter and exit positions quickly, without significant price fluctuations.
Market Participants
Various participants engage in forex trading, each with their own motivations and strategies. The major players in the forex market include commercial banks, central banks, multinational corporations, hedge funds, and retail traders. Central banks play a crucial role in the forex market by implementing monetary policies and managing currency reserves. Commercial banks facilitate most of the forex transactions, both for their clients and for speculative purposes. Multinational corporations engage in forex trading to hedge against currency risks associated with their international operations. Hedge funds and retail traders participate in the market to profit from currency fluctuations.
Currency Pairs
Forex trading involves the simultaneous buying and selling of currencies in pairs. The most commonly traded currency pairs are known as the major pairs, which include the US dollar (USD) paired with currencies such as the euro (EUR), Japanese yen (JPY), British pound (GBP), Swiss franc (CHF), Canadian dollar (CAD), and Australian dollar (AUD). In addition to the major pairs, there are also minor pairs and exotic pairs, which involve currencies from emerging economies.
Market Dynamics
Economic indicators, geopolitical events, central bank policies, and market sentiment are just a few of the many factors that affect the forex market. Economic indicators, such as GDP growth, inflation rates, and employment data, provide insights into the health of a country's economy and can impact the value of its currency. Geopolitical events, such as elections, trade disputes, and natural disasters, can create volatility in the forex market. Central bank policies, such as interest rate decisions and quantitative easing programmes, have a significant impact on currency values. Additionally, investor perceptions and expectations can influence currency movements.
Trading Sessions
Due to its global nature, the forex market operates in different trading sessions, each with its own characteristics. The major trading sessions include the Asian session, the European session, and the North American session. The Asian session, centred around Tokyo, is known for its relatively low volatility. The European session, centred around London, is the most active session, with high liquidity and volatility. The North American session, centred around New York, overlaps with the European session and is characterised by significant trading volume.
Leverage and margin trading
One of the unique features of forex trading is the availability of leverage, which allows traders to control larger positions with a smaller amount of capital. Leverage amplifies both profits and losses, making forex trading a high-risk endeavour. Margin trading is the practice of borrowing funds from a broker to trade larger positions. While leverage and margin trading can enhance potential returns, they also increase the risk of significant losses.
Market Efficiency
The forex market is often considered to be highly efficient due to its size, liquidity, and the participation of numerous market participants. Prices in the forex market reflect all available information and are quickly adjusted to new developments. However, there are instances where inefficiencies arise, creating opportunities for traders to profit. These inefficiencies can be exploited using various trading strategies, including those based on artificial intelligence.
In the next section, we will explore the different AI techniques that can be applied to forex trading, enabling traders to make more informed decisions and potentially improve their trading performance.
AI Techniques for Forex Trading
Artificial intelligence (AI) has revolutionised the way we approach trading in the financial markets. In the context of Forex trading, AI techniques have gained significant popularity due to their ability to analyse vast amounts of data, identify patterns, and make informed trading decisions. In this section, we will explore some of the key AI techniques used in forex trading and their potential benefits and limitations.
Machine Learning in Forex Trading
Machine learning (ML) is a subset of AI that focuses on the development of algorithms and models that can learn from and make predictions or decisions based on data. In forex trading, ML algorithms can be trained on historical market data to identify patterns and trends that can help predict future price movements.
One popular ML technique used in Forex trading is supervised learning, where the algorithm is trained on labelled data, such as historical price data and corresponding indicators. The algorithm learns to recognise patterns and relationships between different variables, enabling it to make predictions on unseen data.
Another ML technique used in Forex trading is unsupervised learning, where the algorithm learns to identify patterns and relationships in the data without any predefined labels. This can be particularly useful in discovering hidden market trends or clustering similar market conditions.
Deep Learning in Forex Trading
Deep learning (DL) is a subset of machine learning that focuses on the development of artificial neural networks capable of learning and making decisions in a manner similar to the human brain. DL algorithms, such as convolutional neural networks (CNNs) and recurrent neural networks (RNNs), have shown great promise in forex trading.
DL algorithms can analyse large amounts of unstructured data, such as news articles, social media sentiment, and even audio or video data, to extract meaningful insights that can influence trading decisions. For example, DL algorithms can analyse news sentiment to gauge market sentiment and make predictions on the impact of news events on currency prices.
Natural Language Processing in Forex Trading
Natural Language Processing (NLP) is a branch of AI that focuses on the interaction between computers and human language. In forex trading, NLP techniques can be used to analyse and understand textual data, such as news articles, economic reports, and social media posts, to extract relevant information that can impact currency prices.
NLP algorithms can perform sentiment analysis to determine the overall sentiment of news articles or social media posts towards a particular currency or economic event. This information can be used to make informed trading decisions, such as predicting the impact of a central bank announcement on currency prices.
Reinforcement Learning in Forex Trading
Reinforcement learning (RL) is a branch of AI that focuses on training agents to make sequential decisions in an environment to maximise a reward. In forex trading, RL algorithms can learn optimal trading strategies by interacting with the market and receiving feedback in the form of profits or losses.
RL algorithms can learn to adapt to changing market conditions and adjust trading strategies accordingly. For example, an RL algorithm can learn to dynamically adjust stop-loss and take-profit levels based on market volatility or optimise trade execution based on slippage and transaction costs.
Benefits of AI Techniques in Forex Trading
The use of AI techniques in forex trading offers several benefits:
Data Analysis: AI algorithms can analyse vast amounts of data quickly and efficiently, enabling traders to identify patterns and trends that may not be apparent to human traders.
Automation: AI algorithms can automate various aspects of trading, such as data collection, analysis, and execution, reducing the need for manual intervention and saving time for traders.
Speed and Efficiency: AI algorithms can process and analyse data in real-time, allowing traders to make faster and more informed trading decisions.
Risk Management: AI algorithms can help traders manage risk by providing insights into market volatility, optimising trade execution, and dynamically adjusting risk parameters.
Adaptability: AI algorithms can adapt to changing market conditions and adjust trading strategies accordingly, improving performance in different market environments.
Limitations of AI Techniques in Forex Trading
While AI techniques offer significant advantages in forex trading, they also have some limitations.
Data Quality: The accuracy and reliability of AI predictions depend on the quality and relevance of the data used for training. Poor-quality or biassed data can lead to inaccurate predictions and trading decisions.
Overfitting: AI algorithms can be prone to overfitting, where they perform well on historical data but fail to generalise to new, unseen data. This can lead to poor performance in live trading.
Lack of Interpretability: Some AI techniques, such as deep learning, can be difficult to interpret and understand. This lack of interpretability can make it challenging for traders to trust and validate the decisions made by AI algorithms.
Dependency on Market Conditions: AI algorithms may perform well in certain market conditions but struggle in others. Traders need to carefully monitor and adapt AI strategies to changing market dynamics.
Ethical Considerations: The use of AI in trading raises ethical concerns, such as the potential for market manipulation or unfair advantage. Traders and regulators need to ensure that AI is used responsibly and in compliance with ethical standards.
AI techniques have the potential to revolutionise Forex trading by enabling traders to analyse vast amounts of data, make informed decisions, and automate various aspects of trading. However, it is essential to understand the benefits and limitations of AI techniques and use them responsibly to enhance trading performance while mitigating risks.
Forex Trading Strategies with AI
Artificial intelligence (AI) has revolutionised the way trading is conducted in the financial markets. In the forex market, AI-powered trading strategies have gained significant popularity due to their ability to analyse vast amounts of data, identify patterns, and make informed trading decisions. In this section, we will explore some of the most effective forex trading strategies that utilise AI techniques.
Trend-following strategies
Trend following is a popular trading strategy that aims to identify and capitalise on the prevailing market trends. AI algorithms can effectively analyse historical price data and identify trends, enabling traders to enter positions in the direction of the trend. These algorithms can adapt to changing market conditions and adjust trading parameters accordingly.
One AI technique commonly used in trend-following strategies is machine learning. Machine learning algorithms can analyse historical price data and identify patterns that indicate the presence of a trend. By training the algorithm on a large dataset, it can learn to recognise different types of trends and generate accurate trading signals.
Another AI technique used in trend-following strategies is deep learning. Deep learning algorithms, such as neural networks, can analyse complex patterns in price data and identify trends with high accuracy. These algorithms can also adapt to changing market conditions and adjust their trading signals accordingly.
Mean Reversion Strategies
Mean reversion is a trading strategy that assumes that prices will eventually revert to their mean or average value. AI techniques can be used to identify potential mean reversion opportunities in the forex market. By analysing historical price data and identifying deviations from the mean, AI algorithms can generate trading signals that indicate when prices are likely to revert.
Machine learning algorithms can be trained to identify mean reversion patterns in price data. By analysing historical price movements and identifying periods of overbought or oversold conditions, these algorithms can generate trading signals that indicate when prices are likely to revert.
Deep learning algorithms can also be used in mean reversion strategies. These algorithms can analyse complex patterns in price data and identify deviations from the mean with high accuracy. By training the algorithm on a large dataset, it can learn to recognise different types of mean reversion patterns and generate accurate trading signals.
Breakout Strategies
Breakout strategies aim to capitalise on significant price movements that occur when prices break through important support or resistance levels. AI techniques can be used to identify potential breakout opportunities in the forex market. By analysing historical price data and identifying patterns that indicate a potential breakout, AI algorithms can generate trading signals that indicate when to enter a position.
Machine learning algorithms can be trained to identify breakout patterns in price data. These algorithms can produce trading signals that show when prices are likely to break through significant levels by analysing historical price movements and identifying consolidation periods followed by a breakout.
Deep learning algorithms can also be used in breakout strategies. These algorithms can analyse complex patterns in price data and identify potential breakout opportunities with high accuracy. By training the algorithm on a large dataset, it can learn to recognise different types of breakout patterns and generate accurate trading signals.
News-Based Strategies
News-based strategies aim to capitalise on market movements that occur as a result of news events. AI techniques, such as natural language processing (NLP), can be used to analyse news articles, social media posts, and other sources of information to identify potential trading opportunities.
NLP algorithms can analyse news articles and extract relevant information, such as economic indicators, corporate announcements, and geopolitical events. By analysing the sentiment and relevance of the news, these algorithms can generate trading signals that indicate when to enter or exit a position.
Hybrid Strategies
Hybrid strategies combine multiple AI techniques to generate trading signals. These strategies aim to leverage the strengths of different AI techniques and improve overall trading performance. For example, a hybrid strategy may combine trend-following and mean reversion techniques to generate more accurate trading signals.
By combining machine learning, deep learning, and NLP techniques, hybrid strategies can analyse a wide range of data sources and generate trading signals that are more robust and reliable.
AI-powered forex trading strategies have the potential to significantly improve trading performance by analysing vast amounts of data, identifying patterns, and generating accurate trading signals. Whether it is trend following, mean reversion, breakout, or news-based strategies, AI techniques can provide traders with a competitive edge in the forex market. However, it is important to note that AI strategies are not foolproof and can still be subject to market risks and uncertainties. Traders should always exercise caution and conduct thorough backtesting and risk management before implementing AI strategies in their trading activities.
Risk Management in Forex Trading
Risk management is a crucial aspect of forex trading, and it becomes even more important when incorporating artificial intelligence (AI) techniques into the trading process. AI can significantly enhance risk management strategies by providing traders with valuable insights, real-time analysis, and automated decision-making capabilities. In this section, we will explore the various ways AI can be utilised for effective risk management in forex trading.
Understanding Risk in Forex Trading
Forex trading involves the buying and selling of currencies in the global foreign exchange market. As with any form of investment, there are inherent risks associated with forex trading. These risks include market volatility, economic factors, geopolitical events, and liquidity risks. Traders need to carefully manage these risks to protect their capital and maximise their potential profits.
AI-Based Risk Assessment
AI can play a vital role in assessing and managing risks in forex trading. By analysing vast amounts of historical and real-time data, AI algorithms can identify patterns, trends, and correlations that may impact currency prices. This analysis enables traders to make informed decisions and implement risk management strategies effectively.
One of the key advantages of AI in risk assessment is its ability to process and analyse data at a speed and scale that is beyond human capabilities. AI algorithms can quickly analyse multiple data sources, including economic indicators, news articles, social media sentiment, and technical indicators. This comprehensive analysis helps traders identify potential risks and take appropriate actions to mitigate them.
Automated Stop-Loss and Take-Profit Orders
Stop-loss and take-profit orders are essential risk management tools in forex trading. These orders automatically close a trade when a specified price level is reached, limiting potential losses or securing profits. AI can automate the process of setting stop-loss and take-profit levels based on predefined rules or through machine learning algorithms.
AI algorithms can analyse historical price data and identify optimal stop-loss and take-profit levels based on market conditions and volatility. By automating these orders, traders can ensure that their risk management strategies are consistently applied, even in fast-moving markets. This automation eliminates the emotional bias that can often lead to poor risk management decisions.
Real-time risk monitoring
AI can provide real-time risk monitoring capabilities, allowing traders to stay updated on market conditions and potential risks. AI algorithms can continuously analyse market data, news events, and other relevant factors to identify any changes that may impact currency prices. Traders can set up alerts or notifications to be informed of significant market movements or potential risk triggers.
Real-time risk monitoring enables traders to react quickly to changing market conditions and adjust their risk management strategies accordingly. By staying informed and proactive, traders can minimise potential losses and maximise their trading opportunities.
AI-Based Portfolio Optimisation
Portfolio optimisation is another critical aspect of risk management in forex trading. AI algorithms can analyse multiple currency pairs and their correlations to identify the optimal portfolio allocation that balances risk and return. By diversifying their portfolio across different currency pairs, traders can reduce the impact of individual currency fluctuations on their overall portfolio performance.
AI can also help in rebalancing the portfolio based on changing market conditions. By continuously analysing market data and portfolio performance, AI algorithms can suggest adjustments to the portfolio allocation to maintain an optimal risk-return profile.
Risk simulation and stress testing
AI can simulate various risk scenarios and perform stress testing to assess the resilience of trading strategies. By simulating different market conditions, AI algorithms can provide traders with insights into the potential risks and rewards associated with their trading strategies. This allows traders to make informed decisions and adjust their strategies accordingly.
Stress testing involves subjecting trading strategies to extreme market conditions to evaluate their performance and resilience. AI algorithms can simulate scenarios such as market crashes, economic downturns, or geopolitical events to assess the impact on the trading strategy. This helps traders identify potential weaknesses and make necessary adjustments to improve risk management.
Incorporating AI into forex trading can significantly enhance risk management strategies. AI algorithms can provide real-time risk assessment, automate stop-loss and take-profit orders, enable real-time risk monitoring, optimise portfolio allocation, and perform risk simulation and stress testing. By leveraging the power of AI, traders can make more informed decisions, minimise potential losses, and maximise their trading opportunities in the forex market. However, it is important to note that AI is not a foolproof solution and should be used in conjunction with human judgement and expertise. Traders should continuously monitor and evaluate the performance of AI-based risk management strategies to ensure their effectiveness in the dynamic forex market.
AI in Stock Trading
Overview of the Stock Market
The stock market is a dynamic and complex financial marketplace where individuals and institutions buy and sell shares of publicly traded companies. It serves as a platform for companies to raise capital and for investors to participate in the growth and success of these companies. The stock market plays a crucial role in the global economy, providing opportunities for wealth creation and capital allocation.
Structure of the Stock Market
The stock market is composed of various exchanges, such as the New York Stock Exchange (NYSE) and the Nasdaq, where stocks are traded. These exchanges act as intermediaries, facilitating the buying and selling of stocks between buyers and sellers. Government organisations, such as the Securities and Exchange Commission (SEC) in the United States, regulate the stock market to ensure fair and open trading practises.
Stocks are categorised into different types based on their characteristics. Common stocks represent ownership in a company and provide voting rights to shareholders. Preferred stocks, on the other hand, offer a fixed dividend payment but do not provide voting rights. Stocks can also be classified based on the size of the company, such as large-cap, mid-cap, and small-cap stocks, which represent companies with different market capitalizations.
Market Participants
The participation of various market participants, each with their own goals and tactics, is what drives the stock market. These participants include individual investors, institutional investors, traders, and market makers.
Individual investors are individuals who buy and sell stocks for their personal investment portfolios. They may include retail investors, who trade through brokerage accounts, and high-net-worth individuals, who have substantial financial resources to invest.
Institutional investors, such as mutual funds, pension funds, and hedge funds, manage large pools of money on behalf of their clients. These investors often have access to extensive resources and employ sophisticated trading strategies.
Traders are individuals or firms that engage in short-term buying and selling of stocks to take advantage of price fluctuations. They aim to profit from the market's volatility by executing trades quickly and frequently.
Market makers are entities that facilitate liquidity in the market by providing continuous bid and ask prices for stocks. They play a crucial role in ensuring smooth trading and narrowing the bid-ask spread.
Market Dynamics
A wide range of factors have an impact on stock prices and market trends in the stock market. These factors include macroeconomic indicators, company-specific news, geopolitical events, and investor sentiment.
Macroeconomic indicators, such as GDP growth, inflation rates, and interest rates, provide insights into the overall health of the economy. Positive economic data can boost investor confidence and drive stock prices higher, while negative data can lead to market downturns.
Company-specific news, such as earnings reports, product launches, and mergers and acquisitions, can significantly impact the stock prices of individual companies. Positive news can attract investors and drive stock prices up, while negative news can lead to sell-offs and price declines.
Geopolitical events, such as trade disputes, political instability, and natural disasters, can create uncertainty in the market and impact investor sentiment. These events can cause market volatility and affect stock prices across various sectors.
Investor sentiment plays a crucial role in shaping market trends. Positive sentiment can lead to bullish market conditions, with investors optimistic about future prospects. Conversely, negative sentiment can result in bearish market conditions, making investors cautious and risk-averse.
Market Efficiency and Anomalies
The stock market is often characterised by the concept of market efficiency, which suggests that stock prices reflect all available information and are difficult to predict consistently. However, the market is not always perfectly efficient, and anomalies can occur.
Anomalies, such as the January effect, the momentum effect, and the value effect, refer to patterns or trends in stock prices that deviate from what would be expected under efficient market conditions. Researchers have extensively studied these anomalies, which has sparked the creation of various trading strategies.
The use of artificial intelligence (AI) in stock trading aims to leverage these anomalies and market inefficiencies to generate profitable trading strategies. By analysing vast amounts of data and identifying patterns, AI algorithms can potentially uncover hidden opportunities and make informed trading decisions.
In the next section, we will explore the different AI techniques used in stock trading and how they can be applied to develop effective trading strategies.
AI Techniques for Stock Trading
Artificial intelligence (AI) has revolutionised the way we approach stock trading. With its ability to analyse vast amounts of data and make predictions based on patterns and trends, AI has become an invaluable tool for traders. In this section, we will explore some of the AI techniques that are commonly used in stock trading.
Machine Learning
Machine learning (ML) is a branch of AI that focuses on the development of algorithms that can learn and make predictions or decisions without being explicitly programmed. In stock trading, ML algorithms can be trained on historical data to identify patterns and trends that can be used to predict future stock prices.
One popular ML technique used in stock trading is supervised learning, where the algorithm is trained on labelled data, meaning it is provided with input data and corresponding output labels. The algorithm learns to map the input data to the correct output labels, allowing it to make predictions on new, unseen data.
Another ML technique used in stock trading is unsupervised learning, where the algorithm is given unlabelled data and tasked with finding patterns or structures within the data. This can be useful for identifying clusters of stocks that exhibit similar behaviour, which can then be used to inform trading strategies.
Deep Learning
Deep learning (DL) is a subset of machine learning that focuses on the development of artificial neural networks inspired by the structure and function of the human brain. These neural networks are capable of learning and making complex decisions based on large amounts of data.
In stock trading, deep learning algorithms, such as convolutional neural networks (CNNs) and recurrent neural networks (RNNs), have been used to analyse stock price data and make predictions. CNNs are particularly effective at analysing time-series data, such as stock prices, by capturing local patterns and dependencies. RNNs, on the other hand, are well-suited for analysing sequential data, such as news articles or social media sentiment, which can impact stock prices.
Natural Language Processing
Natural Language Processing (NLP) is a branch of AI that focuses on the interaction between computers and human language. In stock trading, NLP techniques are used to analyse news articles, social media posts, and other textual data to extract relevant information that can impact stock prices.
Sentiment analysis, a common NLP technique, is used to determine the sentiment expressed in textual data. By analysing the sentiment of news articles or social media posts, traders can gain insights into market sentiment and make informed trading decisions.
Reinforcement Learning
Reinforcement learning (RL) is a branch of AI that focuses on training agents to make decisions in an environment to maximise a reward. In stock trading, RL algorithms can be used to develop trading strategies that maximise profits while minimising risks.
RL algorithms learn through trial and error, receiving feedback in the form of rewards or penalties based on their actions. By continuously interacting with the stock market, RL algorithms can learn optimal trading strategies that adapt to changing market conditions.
Hybrid Approaches
In addition to the individual AI techniques mentioned above, many traders and researchers are exploring hybrid approaches that combine multiple AI techniques to improve trading performance. For example, a hybrid approach may combine machine learning with natural language processing to analyse both historical stock price data and news articles to make more accurate predictions.
By leveraging the strengths of different AI techniques, traders can develop more robust and effective trading strategies.
AI techniques have transformed the field of stock trading, enabling traders to analyse vast amounts of data and make informed decisions. Machine learning, deep learning, natural language processing, and reinforcement learning are just a few of the AI techniques that have been successfully applied to stock trading.
These techniques have the potential to improve trading performance by identifying patterns and trends, predicting stock prices, and managing risks. However, it is important to note that AI is not a magic solution and comes with its own limitations. AI models are only as good as the data they are trained on, and they can be susceptible to biases and overfitting.
As AI continues to evolve, we can expect to see more sophisticated techniques and algorithms being developed for stock trading. Traders who embrace AI and leverage its capabilities will have a competitive edge in the market. However, it is crucial to understand the limitations and potential risks associated with AI in stock trading and to use it as a tool to augment human decision-making rather than replace it entirely.
Stock trading strategies with AI
Artificial intelligence (AI) has revolutionised the way stock trading is conducted. With its ability to analyse vast amounts of data and make predictions based on patterns and trends, AI has become an invaluable tool for traders. In this section, we will explore some of the most effective stock trading strategies that utilise AI.
Trend Following
One popular stock trading strategy that AI excels at is trend-following. This strategy involves identifying and capitalising on the momentum of a stock's price movement. AI algorithms can analyse historical price data and identify patterns that indicate the direction of the trend. By following these trends, traders can enter positions in stocks that are likely to continue their upward or downward movements.
AI-powered trend-following strategies can be highly effective because they can process large amounts of data in real-time and make quick decisions based on the current market conditions. This allows traders to take advantage of short-term price movements and generate profits.
Mean Reversion
Another stock trading strategy that AI can enhance is mean reversion. Mean reversion is based on the concept that stock prices tend to fluctuate around their average value. When a stock deviates significantly from its mean, it is expected to revert back to its average value.
AI algorithms can identify these deviations and predict when a stock is likely to revert to its mean. By entering positions when a stock is oversold or overbought, traders can profit from the price correction that follows.
Mean reversion strategies with AI can be particularly useful in volatile markets where stock prices experience sharp fluctuations. AI algorithms can quickly identify these opportunities and execute trades at the optimal time, maximising potential profits.
Breakout Trading
Breakout trading is a strategy that aims to capitalise on significant price movements that occur when a stock breaks through a support or resistance level. AI algorithms can analyse historical price data and identify patterns that indicate potential breakouts. By entering positions when a breakout occurs, traders can profit from the subsequent price movement.
AI-powered breakout trading strategies can be highly effective because they can quickly identify breakout patterns that may go unnoticed by human traders. Additionally, AI algorithms can continuously monitor multiple stocks and execute trades at the optimal time, increasing the chances of success.
Sentiment Analysis
Sentiment analysis is a stock trading strategy that utilises AI to analyse social media, news articles, and other sources of information to gauge market sentiment. By analysing the sentiment surrounding a particular stock or the overall market, AI algorithms can predict the direction of stock prices.
For example, if there is a positive sentiment surrounding a stock, AI algorithms can identify this and predict that the stock price is likely to increase. Traders can then enter positions in these stocks and profit from the anticipated price movement.
Sentiment analysis with AI can be particularly useful in today's digital age, where information spreads rapidly through social media platforms. By analysing this information in real-time, AI algorithms can provide traders with valuable insights and help them make informed trading decisions.
Portfolio Optimisation
AI can also be used to optimise stock portfolios by selecting the most suitable combination of stocks that maximise returns while minimising risk. AI algorithms can analyse historical data, market trends, and other factors to identify the optimal allocation of assets within a portfolio.
By utilising AI for portfolio optimisation, traders can achieve a more diversified and balanced portfolio, reducing the risk of significant losses. AI algorithms can continuously monitor the performance of the portfolio and make adjustments as market conditions change, ensuring that the portfolio remains optimised.
Automated Trading
One of the most significant advantages of using AI in stock trading is the ability to automate trading processes. AI algorithms can execute trades automatically based on predefined rules and strategies, eliminating the need for manual intervention.
Automated trading with AI offers several benefits. Firstly, it eliminates human emotions from the trading process, ensuring that trades are executed based on objective criteria rather than subjective judgements. Secondly, AI algorithms can process vast amounts of data and execute trades at high speeds, taking advantage of short-term price movements that may go unnoticed by human traders.
Automated trading with AI can be particularly beneficial for high-frequency trading, where trades are executed within milliseconds. AI algorithms can analyse market data and execute trades at lightning-fast speeds, allowing traders to take advantage of small price discrepancies and generate profits.
AI has revolutionised stock trading by providing traders with powerful tools and strategies to make informed decisions. From trend following to mean reversion, breakout trading to sentiment analysis, AI offers a wide range of strategies that can enhance trading performance. Additionally, AI enables portfolio optimisation and automated trading, further improving efficiency and profitability. As AI continues to advance, we can expect even more sophisticated and effective stock trading strategies to emerge, shaping the future of trading.
Risk Management in Stock Trading
Risk management is a crucial aspect of stock trading that ensures the preservation of capital and the mitigation of potential losses. With the integration of artificial intelligence (AI) in stock trading, risk management has become more efficient and effective. AI-powered risk management systems can analyse vast amounts of data, identify patterns, and make informed decisions to minimise risks and maximise returns.
Importance of Risk Management in Stock Trading
Due to the market's sensitivity to a variety of factors, including the economy, world politics, and company-specific news, stock trading is inherently risky. Without proper risk management strategies, traders can expose themselves to significant financial losses. Risk management aims to protect traders from adverse market movements and unexpected events by implementing strategies to control and mitigate risks.
AI in Risk Management
AI has revolutionised risk management in stock trading by providing advanced tools and techniques to analyse and manage risks effectively. Here are some ways AI is used in risk management:
1. Data Analysis and Pattern Recognition
AI algorithms can analyse vast amounts of historical and real-time market data to identify patterns and trends. By recognising patterns, AI systems can predict potential risks and market movements. This enables traders to make informed decisions and adjust their strategies accordingly.
2. Portfolio Optimisation
AI-powered risk management systems can optimise portfolios by considering various risk factors, such as volatility, correlation, and diversification. By analysing these factors, AI algorithms can suggest optimal portfolio allocations that balance risk and return. This helps traders achieve their investment objectives while minimising potential losses.
3. Automated Risk Assessment
AI can automate the process of risk assessment by continuously monitoring market conditions and evaluating the risk exposure of trading positions. AI algorithms can calculate risk metrics, such as value at risk (VaR) and expected shortfall (ES), to provide real-time risk assessments. This allows traders to take proactive measures to mitigate risks and protect their portfolios.
4. Sentiment Analysis
AI-powered sentiment analysis tools can analyse news articles, social media posts, and other sources of information to gauge market sentiment. By understanding market sentiment, traders can assess the potential impact of positive or negative news on stock prices. This helps in making informed decisions and managing risks associated with market sentiment.
5. Machine Learning for Risk Prediction
Machine learning algorithms can learn from historical data to predict future risks and market movements. By analysing patterns and correlations, AI systems can identify potential risks and provide early warnings. This enables traders to take preventive action and adjust their strategies accordingly.
Benefits of AI in Risk Management
The integration of AI in risk management offers several benefits to stock traders:
1. Enhanced Risk Assessment
AI-powered risk management systems can provide more accurate and comprehensive risk assessments compared to traditional methods. By analysing vast amounts of data and considering multiple risk factors, AI algorithms can identify potential risks that may go unnoticed by human traders. This helps in making more informed decisions and reducing the likelihood of unexpected losses.
2. Real-time monitoring
AI algorithms can continuously monitor market conditions and assess the risk exposure of trading positions in real-time. This allows traders to respond quickly to changing market dynamics and take the necessary actions to mitigate risks. Real-time monitoring helps in minimising losses and maximising returns by adapting to market conditions promptly.
3. Improved decision-making
AI-powered risk management systems provide traders with valuable insights and recommendations based on data analysis and pattern recognition. By leveraging AI algorithms, traders can make more informed decisions and adjust their strategies to minimise risks. This improves the overall decision-making process and increases the probability of successful trades.
4. Automation and Efficiency
AI automates various risk management tasks, such as data analysis, risk assessment, and portfolio optimisation. This reduces the manual effort required for risk management and improves efficiency. Traders can focus on strategic decision-making while AI algorithms handle routine risk management tasks. Automation also reduces the chances of human errors and ensures consistent risk management practices.
Limitations of AI in Risk Management
While AI offers significant advantages in risk management, there are also limitations that traders should be aware of:
1. Overreliance on historical data
AI algorithms rely on historical data to make predictions and assess risks. However, market conditions can change rapidly, and historical data may not always reflect current market dynamics accurately. Traders should be cautious about overreliance on historical data and consider other factors, such as real-time market information and qualitative analysis, to make well-informed decisions.
2. Lack of human judgement
AI algorithms are based on mathematical models and patterns, which may not always capture the full complexity of the market. Human judgement and intuition play a crucial role in risk management, especially in situations where unexpected events or market anomalies occur. Traders should use AI as a tool to support their decision-making process rather than relying solely on AI-generated recommendations.
3. Data Quality and Bias
The accuracy and reliability of AI algorithms depend on the quality and diversity of the data used for training. Biased or incomplete data can lead to inaccurate predictions and risk assessments. Traders should ensure that the data used for AI analysis is of high quality and representative of market conditions. Regular monitoring and validation of AI models are essential to identify and address any biases or data quality issues.
4. Regulatory and Ethical Considerations
The use of AI in risk management raises regulatory and ethical considerations. Traders should ensure compliance with applicable regulations and consider the ethical implications of using AI algorithms for risk management. Transparency, explain ability, and fairness in AI decision-making are important factors to consider when implementing AI-powered risk management systems.
AI has transformed risk management in stock trading by providing advanced tools and techniques to analyse and manage risks effectively. AI-powered risk management systems offer enhanced risk assessment, real-time monitoring, improved decision-making, and automation. However, traders should be aware of the limitations of AI, such as overreliance on historical data, lack of human judgement, data quality and bias issues, and regulatory and ethical considerations. By leveraging the benefits of AI while considering its limitations, traders can effectively manage risks and increase their chances of success in stock trading.
Ethical and Legal Considerations of AI in Trading
Ethical Issues in AI Trading
As the use of artificial intelligence (AI) in trading stocks and forex continues to grow, it is important to consider the ethical implications that arise from this technology. While AI has the potential to revolutionise the trading industry by improving efficiency and accuracy, it also raises concerns regarding fairness, transparency, and accountability. In this section, we will explore some of the key ethical issues associated with AI trading.
Fairness and bias
One of the primary ethical concerns in AI trading is the potential for bias in decision-making algorithms. Historical data may contain biases that the algorithms unintentionally learn and perpetuate when training AI systems. This can lead to unfair treatment of certain market participants or the perpetuation of existing inequalities. For example, if an AI trading system is trained on data that reflects biased trading practices, it may inadvertently discriminate against certain groups or favour specific market participants.
To address this issue, it is crucial to ensure that the training data used for AI trading systems is diverse, representative, and free from biases. Additionally, ongoing monitoring and evaluation of AI systems should be conducted to identify and mitigate any biases that may arise during their operation.
Transparency and explainability
Another ethical concern in AI trading is the lack of transparency and explainability of AI algorithms. Many AI models, such as deep learning neural networks, are often considered "black boxes" because they make decisions based on complex patterns and relationships that are difficult for humans to understand. This lack of transparency can make it challenging to determine how and why certain trading decisions are made, which can undermine trust in the system.
To address this issue, efforts should be made to develop AI trading systems that are more transparent and explainable. This can be achieved through the use of interpretable AI models or by providing users with clear explanations of the factors and variables that influence the system's decision-making process. By increasing transparency and explainability, market participants can have a better understanding of how AI systems operate and make informed decisions.
Privacy and data security
AI trading systems rely heavily on vast amounts of data, including personal and sensitive information about market participants. This raises concerns about privacy and data security. Market participants may be hesitant to share their personal information with AI systems, fearing that it may be misused or compromised. Additionally, there is a risk of data breaches or unauthorised access to sensitive trading data, which can have severe financial and reputational consequences.
To address these concerns, it is essential to implement robust data protection measures and adhere to strict privacy regulations. AI trading systems should only collect and store the necessary data for their operation, and appropriate safeguards should be in place to protect the confidentiality and integrity of the data. Market participants should also be provided with clear information about how their data will be used and have the option to opt out if they have concerns about privacy.
Market manipulation and unfair advantage
The use of AI in trading has the potential to enable market manipulation and provide unfair advantages to certain market participants. AI algorithms can analyse vast amounts of data and execute trades at high speeds, giving them the ability to exploit market inefficiencies or engage in predatory trading practices. This can lead to market manipulation, where AI systems manipulate prices or create artificial demand to benefit their owners or operators.
To prevent market manipulation and ensure a level playing field, regulatory bodies need to establish clear guidelines and regulations for the use of AI in trading. These regulations should address issues such as high-frequency trading, algorithmic trading strategies, and the use of AI for market manipulation. By implementing appropriate regulations, market integrity can be maintained, and the risk of unfair advantage can be minimised.
Job Displacement and Economic Impact
The widespread adoption of AI in trading may also have significant economic and social implications. While AI can improve efficiency and reduce costs, it may also lead to job displacement and unemployment in the trading industry. As AI systems become more advanced, they may be able to take over tasks that previously required human traders, which could result in job losses.
To mitigate the impact of job displacement, it is crucial to invest in retraining and upskilling programmes for traders and other market participants. By equipping individuals with the necessary skills to work alongside AI systems, they can adapt to the changing landscape of the trading industry and find new opportunities for employment.
The use of AI in trading stocks and forex brings numerous benefits, but it also raises ethical concerns that need to be addressed. Fairness, transparency, privacy, market manipulation, and job displacement are among the key ethical issues associated with AI trading. By implementing appropriate regulations, promoting transparency and explainability, and prioritising privacy and data security, the ethical challenges of AI trading can be effectively managed. It is essential for market participants, regulators, and AI developers to work together to ensure that AI is used responsibly and ethically in the trading industry.
Regulatory Framework for AI in Trading
As the use of artificial intelligence (AI) in trading stocks and forex continues to grow, it is essential to establish a regulatory framework to ensure the ethical and responsible use of this technology. The rapid advancement of AI has raised concerns about potential risks and challenges that need to be addressed to protect investors and maintain market integrity. In this section, we will explore the regulatory considerations and frameworks that are emerging to govern the use of AI in trading.
Regulatory Challenges
The integration of AI into trading introduces several regulatory challenges. One of the primary concerns is the potential for market manipulation. AI algorithms can analyse vast amounts of data and execute trades at high speeds, which could be exploited to manipulate prices or create artificial market conditions. Regulators need to develop mechanisms to detect and prevent such manipulative practices.
Another challenge is the need for transparency and explainability. AI algorithms often operate as black boxes, making it difficult to understand the decision-making process behind their trading strategies. This lack of transparency raises concerns about accountability and the ability to identify and address potential biases or errors in the algorithms. Regulators must establish guidelines to ensure that AI systems are transparent and explainable, allowing for effective oversight and risk management.
Additionally, data privacy and security are critical considerations. AI algorithms rely on vast amounts of data, including personal and sensitive information. Regulators must ensure that the collection, storage, and use of data comply with privacy regulations to protect individuals' rights and prevent unauthorised access or misuse of data.
Current regulatory efforts
Regulators around the world are actively working to address the regulatory challenges posed by AI in trading. Several initiatives and frameworks have been proposed or implemented to provide guidelines and oversight for the use of AI in financial markets.
One example is the European Union's General Data Protection Regulation (GDPR), which sets strict rules for the collection, processing, and storage of personal data. The GDPR aims to protect individuals' privacy rights and ensure that AI systems comply with data protection regulations. Companies using AI in trading must adhere to these regulations to safeguard customer data and maintain trust.
Another notable effort is the Financial Stability Board's (FSB) work on AI and machine learning in financial markets. The FSB has established a framework that focuses on the governance and explainability of AI systems. It emphasises the need for robust risk management, model validation, and human oversight to ensure the responsible use of AI in trading.
Furthermore, regulatory bodies such as the U.S. Securities and Exchange Commission (SEC) and the Financial Conduct Authority (FCA) in the UK have been actively monitoring and addressing the use of AI in trading. They have issued guidelines and warnings to market participants, emphasising the importance of compliance with existing regulations and the need for transparency and accountability in AI-driven trading strategies.
Future Regulatory Considerations
As AI technology continues to evolve, regulators must adapt and update their frameworks to address emerging challenges. Here are some key considerations for future regulatory efforts:
Risk-based approach: Regulators should adopt a risk-based approach to assess the potential risks associated with AI in trading. This approach would involve identifying and prioritising the most significant risks and developing appropriate regulatory measures to mitigate them.
International cooperation: Given the global nature of financial markets, international cooperation among regulators is crucial. Collaboration and information sharing can help establish consistent regulatory standards and prevent regulatory arbitrage.
Testing and validation: Regulators should encourage the development and adoption of standardised testing and validation procedures for AI algorithms used in trading. This would ensure that algorithms are thoroughly tested for accuracy, reliability, and compliance with regulatory requirements.
Ethical guidelines: Regulators should consider developing ethical guidelines for the use of AI in trading. These guidelines would address issues such as fairness, transparency, and accountability, ensuring that AI systems are designed and used in a manner that aligns with societal values.
Ongoing monitoring and evaluation: Regulators should establish mechanisms for ongoing monitoring and evaluation of AI systems in trading. This would involve periodic assessments of the impact of AI on market dynamics, investor protection, and market integrity.
The regulatory framework for AI in trading is still evolving as regulators grapple with the challenges and opportunities presented by this technology. Establishing effective regulations is crucial to ensuring the responsible and ethical use of AI in financial markets. By addressing concerns related to market manipulation, transparency, data privacy, and security, regulators can foster trust and confidence in AI-driven trading systems. Ongoing collaboration and international cooperation will be essential to developing consistent regulatory standards that protect investors and maintain market integrity in the future.
Transparency and Explainability in AI Trading
Transparency and explainability are crucial aspects when it comes to the use of artificial intelligence (AI) in trading stocks and forex. As AI algorithms become more sophisticated and complex, it becomes increasingly important to understand how they make decisions and to ensure that these decisions are transparent and explainable.
The Importance of Transparency
Transparency refers to the ability to understand and explain the decision-making process of AI algorithms. In the context of trading, transparency is essential for several reasons. Firstly, it allows traders and investors to have confidence in the AI systems they are using. When the decision-making process is transparent, users can understand why a particular trade was executed or why a certain prediction was made.
Secondly, transparency is crucial for regulatory compliance. Financial markets are heavily regulated, and regulators require transparency to ensure that trading activities are fair and in compliance with the law. Without transparency, it becomes difficult to assess whether AI systems are operating within the boundaries set by regulators.
Thirdly, transparency is important for risk management. Traders need to be able to assess the risks associated with using AI systems and understand the potential impact of these systems on their portfolios. Transparent AI systems allow traders to identify and mitigate potential risks more effectively.
The Challenge of Explainability
Explainability refers to the ability to provide understandable explanations for the decisions made by AI algorithms. Explainability goes beyond transparency by offering succinct, human-friendly explanations that are easy to understand.
Explainability is particularly challenging in the context of AI trading systems. Many AI algorithms, such as deep learning neural networks, are highly complex and operate as "black boxes." They can make accurate predictions, but it is often difficult to understand how they arrived at those predictions.
The lack of explainability in AI trading systems raises concerns for traders, investors, and regulators. Without clear explanations, it becomes challenging to trust the decisions made by AI algorithms. Traders may be hesitant to rely on AI systems if they cannot understand the reasoning behind the recommendations or predictions.
Addressing transparency and explainability
To address the challenges of transparency and explainability in AI trading, several approaches can be taken:
Interpretable AI Models: Researchers are actively working on developing AI models that are more interpretable. These models aim to provide clear explanations for their decisions, allowing traders and investors to understand the underlying reasoning. Techniques such as rule-based systems and decision trees can provide more transparent and explainable models.
Model Documentation: It is essential to document the AI models used in trading systems thoroughly. This documentation should include information about the model architecture, training data, and any pre-processing steps. By providing detailed documentation, traders and regulators can gain insights into how the AI system operates.
Algorithmic Auditing: Regular audits of AI trading systems can help ensure transparency and explainability. Audits involve reviewing the decision-making process, analysing the inputs and outputs of the AI system, and assessing its performance. By conducting audits, traders can gain a better understanding of how the AI system is making decisions and identify any potential biases or issues.
Regulatory Oversight: Regulators play a crucial role in ensuring transparency and explainability in AI trading. They can establish guidelines and standards for AI systems used in trading and require companies to provide transparency reports. Regulators can also conduct independent audits to verify the fairness and compliance of AI trading systems.
Ethical Considerations: Transparency and explainability are closely tied to ethical considerations in AI trading. Traders and developers should prioritise ethical practices and ensure that AI systems are not biassed or discriminatory. By addressing ethical concerns, transparency and explainability can be enhanced.
Benefits of Transparency and Explainability
Transparency and explainability in AI trading offer several benefits:
Trust and Confidence: When traders and investors can understand the decision-making process of AI systems, they are more likely to trust and have confidence in those systems. This can lead to increased adoption of AI trading technologies.
Regulatory Compliance: Transparent and explainable AI systems help traders and companies comply with regulatory requirements. By providing clear explanations for trading decisions, companies can demonstrate that they are operating within the boundaries set by regulators.
Risk Management: Transparent AI systems allow traders to assess and manage risks more effectively. By understanding the reasoning behind AI recommendations, traders can make more informed decisions and mitigate potential risks.
Improved Performance: Transparency and explainability can lead to improved performance in AI trading systems. When traders can understand the decision-making process, they can identify and address any weaknesses or biases in the system, leading to better overall performance.
Challenges and limitations
While transparency and explainability are essential, there are challenges and limitations to achieving them in AI trading.
Complexity of AI Algorithms: Many AI algorithms used in trading, such as deep learning neural networks, are highly complex and operate as black boxes. It can be challenging to provide clear explanations for the decisions made by these algorithms.
Trade-Off with Performance: Increasing transparency and explainability may come at the cost of performance. Some AI models that are more interpretable may not achieve the same level of accuracy as more complex models.
Data Privacy and Security: Providing transparency and explainability may require sharing sensitive trading data, raising concerns about data privacy and security. It is crucial to strike a balance between transparency and protecting sensitive information.
Regulatory Challenges: Establishing regulatory frameworks for transparency and explainability in AI trading can be challenging. Regulators need to keep up with the rapid advancements in AI technology and ensure that regulations are effective and enforceable.
Transparency and explainability are critical considerations in the use of AI in trading stocks and forex. They are essential for building trust, ensuring regulatory compliance, and managing risks effectively. While there are challenges and limitations, ongoing research and regulatory efforts are focused on addressing these issues and enhancing transparency and explainability in AI trading systems.
Bias and Fairness in AI Trading
As artificial intelligence (AI) continues to revolutionise the trading industry, it is crucial to address the issue of bias and fairness in AI trading systems. Bias refers to systematic favouritism or discrimination towards certain groups or individuals, while fairness refers to the absence of bias and the equitable treatment of all market participants. In the context of AI trading, bias and fairness are essential considerations to ensure ethical and transparent practices.
Understanding Bias in AI Trading
Bias in AI trading can arise from various sources, including biased data, biased algorithms, and biased decision-making processes. Biased data refers to the input data used to train AI models, which may contain inherent biases due to historical patterns or human prejudices. Biased algorithms can result from the design and implementation of AI models that inadvertently favour certain outcomes or groups. Biased decision-making processes occur when AI systems make decisions that disproportionately impact certain market participants or perpetuate existing inequalities.
The Impact of Bias in AI Trading
The presence of bias in AI trading systems can have significant consequences. Firstly, biased AI models can lead to unfair trading practises where certain market participants are disadvantaged or excluded from opportunities. This can undermine market integrity and erode trust in the financial system. Secondly, biased trading algorithms can amplify existing market inequalities, exacerbating disparities in wealth distribution and access to financial resources. Lastly, biased decision-making processes can result in unintended consequences, such as market volatility or systemic risks.
Ensuring Fairness in AI Trading
To address bias and promote fairness in AI trading, several measures can be implemented. Firstly, it is crucial to ensure diverse and representative data sets for training AI models. By incorporating data from a wide range of sources and perspectives, the risk of bias can be minimised. Additionally, ongoing monitoring and evaluation of AI models can help identify and rectify any biases that may emerge over time. Regular audits and reviews of AI trading systems can provide transparency and accountability, ensuring that biases are detected and corrected promptly.
Another approach to ensuring fairness in AI trading is through the use of explainable AI (XAI) techniques. XAI aims to provide clear and interpretable explanations for the decisions made by AI models. By understanding the underlying factors and reasoning behind AI-generated trading decisions, market participants can assess the fairness and potential biases in the system. XAI can also facilitate regulatory compliance and enable market participants to challenge or contest AI-generated decisions if necessary.
Challenges in Achieving Fairness
While the goal of achieving fairness in AI trading is essential, it is not without its challenges. One significant challenge is the inherent complexity of AI models and algorithms. AI systems often operate as black boxes, making it difficult to understand the underlying decision-making processes. This lack of transparency can hinder efforts to identify and address biases effectively. Additionally, biases can be subtle and difficult to detect, requiring sophisticated techniques and continuous monitoring to ensure fairness.
Another challenge is the potential for unintended consequences when attempting to eliminate bias. In some cases, efforts to remove bias from AI models may inadvertently introduce new biases or distort the system's performance. Striking the right balance between fairness and performance is a delicate task that requires careful consideration and ongoing refinement.
The Role of Regulation
Regulatory frameworks play a crucial role in addressing bias and ensuring fairness in AI trading. Regulators can establish guidelines and standards for the development and deployment of AI trading systems, emphasising the importance of fairness and transparency. They can also require market participants to conduct regular audits and assessments of their AI systems to detect and rectify biases. Additionally, regulators can encourage the adoption of XAI techniques to enhance transparency and accountability in AI trading.
Bias and fairness are critical considerations in the future of AI trading. As AI continues to shape the trading landscape, it is essential to address biases that may arise from data, algorithms, and decision-making processes. By ensuring diverse and representative data sets, implementing explainable AI techniques, and establishing robust regulatory frameworks, we can strive towards a more fair and equitable trading environment. Achieving fairness in AI trading is an ongoing journey that requires collaboration between market participants, regulators, and AI developers to build trust and confidence in the financial markets.
The Future of AI in Trading
Emerging Trends in AI Trading
Artificial intelligence (AI) has revolutionised the trading industry, enabling traders to make more informed decisions and execute trades with greater efficiency. As technology continues to advance, new trends are emerging in AI trading that are shaping the future of the industry. In this section, we will explore some of these emerging trends and their potential impact on the trading landscape.
1. Machine learning and deep learning
Machine learning (ML) and deep learning (DL) are two of the most prominent trends in AI trading. ML algorithms analyse vast amounts of historical data to identify patterns and make predictions about future market movements. DL, a subset of ML, uses artificial neural networks to process complex data and extract meaningful insights. These techniques have the potential to enhance trading strategies by identifying hidden patterns and generating more accurate predictions.
One emerging trend in ML and DL is the use of generative models, such as generative adversarial networks (GANs), to create synthetic financial data. These models can generate realistic market scenarios, allowing traders to test their strategies in simulated environments before deploying them in real markets. This trend has the potential to revolutionise backtesting and strategy development, enabling traders to refine their approaches and improve their performance.
2. Natural Language Processing (NLP)
NLP is another emerging trend in AI trading. It involves the analysis of textual data, such as news articles, social media posts, and earnings reports, to extract valuable insights that can inform trading decisions. NLP techniques can be used to gauge market sentiment, identify emerging trends, and assess the impact of news events on financial markets.
One exciting development in NLP is the use of sentiment analysis to predict market movements. By analysing the sentiment of news articles and social media posts, AI algorithms can gauge the overall market sentiment and make predictions about future price movements. This trend has the potential to provide traders with valuable real-time insights and improve their decision-making process.
3. Reinforcement Learning
Reinforcement learning (RL) is a branch of AI that focuses on training algorithms to make decisions based on trial and error. RL algorithms learn from their actions and receive feedback in the form of rewards or penalties, enabling them to optimise their strategies over time. In the context of trading, RL can be used to develop autonomous trading agents that learn to navigate the market and maximise profits.
An emerging trend in RL is the use of multi-agent systems, where multiple RL agents interact with each other and the market. These agents can collaborate or compete with each other, leading to the emergence of complex trading strategies and behaviours. This trend has the potential to create more dynamic and adaptive trading systems that can quickly respond to changing market conditions.
4. Quantum Computing
Quantum computing is an emerging technology that has the potential to revolutionise AI trading. Quantum computers leverage the principles of quantum mechanics to perform complex calculations at an unprecedented speed. In the context of trading, quantum computing can be used to optimise portfolio allocation, solve complex optimisation problems, and simulate market scenarios.
One of the most significant advantages of quantum computing in trading is its ability to perform parallel computations. This means that quantum computers can process multiple trading strategies simultaneously, enabling traders to explore a vast solution space and identify optimal trading approaches. However, quantum computing is still in its early stages, and its practical implementation in trading is yet to be fully realised.
5. Explainable AI
Explainable AI is an emerging trend that focuses on making AI models more transparent and interpretable. In the trading industry, where decisions can have significant financial implications, it is crucial to understand how AI algorithms arrive at their predictions. Explainable AI techniques aim to provide traders with insights into the decision-making process of AI models, enabling them to validate the results and identify potential biases or errors.
One approach to explainable AI in trading is the use of rule-based systems that generate trading signals based on predefined rules and logic. These systems provide clear explanations for their decisions, making it easier for traders to understand and trust the AI-generated signals. Another approach is the use of model-agnostic techniques, such as LIME (Local Interpretable Model-Agnostic Explanations), which provide explanations for the predictions of any black-box model.
The future of AI in trading is filled with exciting possibilities. Emerging trends such as machine learning, deep learning, natural language processing, reinforcement learning, quantum computing, and explainable AI are reshaping the way traders analyse and execute trades. These trends have the potential to enhance trading strategies, improve decision-making processes, and ultimately drive better financial outcomes. However, it is important to acknowledge the challenges and ethical considerations associated with the use of AI in trading, as discussed in the previous chapters. As technology continues to evolve, it is crucial for traders and regulators to stay informed and adapt to the changing landscape of AI in trading.
Challenges and opportunities
As the use of artificial intelligence (AI) in trading stocks and forex continues to evolve, it brings with it a set of challenges and opportunities. While AI has the potential to revolutionise the way trading is conducted, it also presents certain obstacles that need to be addressed. In this section, we will explore some of the key challenges and opportunities associated with the future of AI in trading.
Challenges
1. Data Quality and Availability
One of the primary challenges in using AI for trading is the quality and availability of data. AI algorithms rely heavily on large volumes of high-quality data to make accurate predictions and decisions. However, financial data can be complex, noisy, and prone to errors. Ensuring the accuracy and reliability of data is crucial for the success of AI trading systems. Additionally, accessing real-time and historical data can be a challenge, especially for smaller market participants who may not have the resources to gather and maintain extensive datasets.
2. Model interpretability and explainability
Another challenge is the interpretability and explainability of AI models. Deep learning algorithms, for example, are often considered black boxes, making it difficult to understand how they arrive at their predictions. This lack of transparency can be a concern for regulators, investors, and traders who need to understand the reasoning behind AI-driven trading decisions. Developing techniques to make AI models more interpretable and explainable is an ongoing area of research.
3. Overfitting and generalisation
Overfitting is a common challenge in AI trading systems. Overfitting occurs when a model performs well on historical data but fails to generalise to new, unseen data. This can lead to poor performance and unreliable trading strategies. Balancing the complexity of AI models to avoid overfitting while still capturing meaningful patterns in the data is a delicate task. Regularisation techniques and robust validation procedures are employed to mitigate the risk of overfitting.
4. Ethical and Legal Considerations
The use of AI in trading raises ethical and legal considerations. AI algorithms can potentially manipulate markets, exploit vulnerabilities, or engage in unethical practices. Regulators are increasingly focusing on the ethical implications of AI in trading and are working to establish guidelines and regulations to ensure fair and transparent markets. Addressing these ethical and legal concerns is crucial for the widespread adoption of AI in trading.
Opportunities
1. Enhanced Decision-Making
AI has the potential to significantly enhance decision-making in trading. By analysing vast amounts of data and identifying patterns that may not be apparent to human traders, AI algorithms can generate more accurate predictions and make informed trading decisions. This can lead to improved profitability and risk management for traders and investors.
2. Automation and Efficiency
AI can automate various aspects of trading, leading to increased efficiency and reduced human error. Tasks such as data collection, analysis, and trade execution can be automated, freeing up traders' time to focus on higher-level strategic decisions. Automated trading systems can operate 24/7, taking advantage of market opportunities that may arise outside of regular trading hours.
3. Risk Management and Compliance
AI can play a crucial role in risk management and compliance in trading. By continuously monitoring market conditions and analysing data in real-time, AI algorithms can identify potential risks and anomalies, allowing traders to take proactive measures to mitigate them. Additionally, AI can help ensure compliance with regulatory requirements by automating the monitoring and reporting of trading activities.
4. Market Efficiency and Liquidity
The use of AI in trading has the potential to improve market efficiency and liquidity. AI algorithms can quickly process vast amounts of information and react to market events in real-time, leading to faster and more efficient price discovery. This can result in narrower bid-ask spreads, increased liquidity, and reduced transaction costs for market participants.
5. Innovation and adaptability
AI opens up new avenues for innovation in trading. As technology continues to advance, new AI techniques and algorithms are being developed, allowing traders to explore novel trading strategies and approaches. AI also enables traders to adapt to changing market conditions more effectively by quickly analysing and responding to new information.
The future of AI in trading stocks and forex presents both challenges and opportunities. Overcoming the challenges related to data quality, model interpretability, overfitting, and ethical considerations is crucial for the successful integration of AI in trading. However, the opportunities for enhanced decision-making, automation, risk management, market efficiency, and innovation make AI a promising tool for traders and investors. As the field continues to evolve, it is essential to strike a balance between harnessing the power of AI and addressing the associated challenges to ensure a fair, transparent, and efficient trading environment.
Impact on Financial Markets
The integration of artificial intelligence (AI) into the world of trading has had a significant impact on financial markets. AI technologies have revolutionised the way trading is conducted, providing traders with powerful tools and insights to make more informed decisions. This section explores the impact of AI on financial markets, highlighting both the positive and negative aspects.
Increased efficiency and speed
One of the most significant impacts of AI in trading is the increased efficiency and speed at which transactions are executed. AI algorithms can analyse vast amounts of data in real-time, identifying patterns and trends that may not be apparent to human traders. This enables traders to make faster and more accurate decisions, leading to improved trading outcomes.
Furthermore, AI-powered trading systems can automate various tasks, such as data collection, analysis, and execution of trades. This automation eliminates the need for manual intervention, reducing the potential for human error and increasing the overall efficiency of trading operations.
Enhanced Market Analysis
AI technologies have greatly enhanced market analysis capabilities in financial markets. Machine learning algorithms can process large volumes of historical and real-time data, identifying complex patterns and relationships. This enables traders to gain deeper insights into market dynamics and make more informed predictions about future price movements.
Additionally, AI-powered trading systems can perform advanced technical and fundamental analysis, incorporating a wide range of indicators and factors. This comprehensive analysis allows traders to identify potential trading opportunities and develop more effective strategies.
Improved risk management
AI has also had a significant impact on risk management in financial markets. AI algorithms can assess and analyse various risk factors, such as market volatility, liquidity, and correlation between assets. This enables traders to better understand and manage their exposure to risk, leading to more effective risk mitigation strategies.
Furthermore, AI-powered trading systems can continuously monitor market conditions and adjust trading strategies accordingly. This dynamic risk management approach helps traders adapt to changing market conditions and reduce the potential for significant losses.
Increased market liquidity
The integration of AI into trading has also contributed to increased market liquidity. AI algorithms can execute trades at high speeds and in large volumes, providing liquidity to the market. This liquidity benefits all market participants, as it reduces bid-ask spreads and improves the overall efficiency of price discovery.
Moreover, AI-powered trading systems can identify and exploit arbitrage opportunities across different markets and asset classes. By capitalising on these opportunities, AI traders contribute to the overall efficiency and liquidity of financial markets.
Potential for Market Disruptions
While AI has brought numerous benefits to financial markets, it also poses certain risks and challenges. One of the concerns is the potential for market disruptions caused by AI-driven trading strategies. The speed and automation of AI algorithms can lead to rapid and large-scale trading activities, which may amplify market volatility and increase the risk of flash crashes.
Additionally, the reliance on AI algorithms for trading decisions introduces the risk of algorithmic errors or malfunctions. A single error in an AI-driven trading system can have significant consequences, potentially leading to substantial financial losses or market disruptions.
Impact on Employment
The widespread adoption of AI in trading has raised concerns about its impact on employment in the financial industry. Trade execution and data analysis are just two examples of the tasks that AI-powered trading systems can handle. This automation has the potential to reduce the demand for human traders and other related roles.
However, it is important to note that AI also creates new job opportunities in areas such as AI development, algorithm design, and system maintenance. Additionally, human traders can leverage AI technologies to enhance their decision-making processes and improve their overall performance.
Regulatory Challenges
The integration of AI in trading has presented regulatory challenges for financial authorities. The complex nature of AI algorithms and their potential impact on market dynamics require regulators to develop appropriate frameworks to ensure market integrity and investor protection.
Regulatory bodies are tasked with addressing issues such as algorithmic trading, market manipulation, and fairness in AI-driven trading. Striking the right balance between fostering innovation and maintaining market stability is crucial to harnessing the full potential of AI in trading.
The impact of AI on financial markets has been profound. It has brought increased efficiency, enhanced market analysis capabilities, improved risk management, and increased market liquidity. However, it also poses risks, such as potential market disruptions and challenges related to employment and regulation.
As AI technologies continue to evolve, it is essential for market participants and regulators to adapt and embrace these advancements responsibly. By leveraging the benefits of AI while addressing its challenges, the future of trading in stocks and forex can be shaped in a way that maximises efficiency, transparency, and fairness in financial markets.
Predictions for the Future
As we look ahead to the future of AI in trading stocks and forex, it is clear that this technology will continue to play a significant role in shaping the financial markets. The advancements in AI and machine learning algorithms have already revolutionised the way trading is conducted, and we can expect further developments in the coming years. In this section, we will explore some predictions for the future of AI in trading and discuss the potential implications.
1. Increased Adoption of AI in Trading
One of the most significant predictions for the future is the increased adoption of AI in trading. As the technology continues to mature and demonstrate its effectiveness, more financial institutions and individual traders will embrace AI-powered trading systems. The ability of AI algorithms to analyse vast amounts of data, identify patterns, and make informed trading decisions in real-time provides a competitive advantage in the fast-paced world of financial markets.
2. Enhanced Trading Strategies
With the advancements in AI techniques, we can expect to see more sophisticated and effective trading strategies being developed. Machine learning algorithms will continue to evolve, enabling traders to uncover hidden patterns and correlations in market data that were previously difficult to detect. This will lead to the development of more accurate predictive models and trading systems that can adapt to changing market conditions.
3. Improved Risk Management
AI will also play a crucial role in improving risk management in trading. By analysing historical data and market trends, AI algorithms can identify potential risks and provide traders with valuable insights to make informed decisions. This will help traders mitigate risks and minimise losses, ultimately leading to more successful and profitable trading strategies.
4. Integration of AI with Other Technologies
In the future, we can expect to see the integration of AI with other emerging technologies, such as blockchain and the Internet of Things (IoT). This integration will enable real-time data collection and analysis, allowing traders to make more accurate predictions and execute trades with greater efficiency. The combination of AI, blockchain, and IoT will create a more transparent and secure trading environment.
5. Personalised Trading Solutions
As AI technology becomes more advanced, we can anticipate the development of personalised trading solutions tailored to individual traders' preferences and risk tolerance. AI algorithms will be able to learn from traders' behaviour and adapt their strategies accordingly, providing personalised recommendations and trade execution. This level of customization will empower traders to achieve their financial goals more effectively.
6. Ethical and Regulatory Challenges
While the future of AI in trading holds great promise, it also presents ethical and regulatory challenges. As AI algorithms become more autonomous and make decisions without human intervention, questions regarding accountability and transparency arise. Regulators will need to establish frameworks to ensure that AI-powered trading systems operate within ethical boundaries and comply with existing regulations. Additionally, efforts to address bias and fairness in AI algorithms will be crucial to maintaining market integrity.
7. Job Displacement and Skill Requirements
The increasing adoption of AI in trading may lead to job displacement in certain areas of the financial industry. As AI algorithms automate tasks traditionally performed by humans, there may be a shift in the skill requirements for traders and financial professionals. The ability to understand and interpret AI-generated insights, as well as the capacity to develop and fine-tune AI models, will become essential skills for traders in the future.
8. Continued Research and Development
The future of AI in trading will heavily rely on continued research and development. As new challenges and opportunities arise, researchers and industry professionals will need to collaborate to enhance AI algorithms, improve data analysis techniques, and address ethical and regulatory concerns. The financial industry will continue to invest in AI research and development to stay at the forefront of technological advancements.
The future of AI in trading stocks and forex is promising. We can expect increased adoption of AI, enhanced trading strategies, improved risk management, and the integration of AI with other technologies. However, ethical and regulatory challenges, job displacement, and the need for continued research and development are important considerations. As AI technology continues to evolve, it is crucial for traders, financial institutions, and regulators to work together to harness its potential while ensuring market integrity and fairness.
TradeFxP Features
If you choose to be a self-employed retail trader, here are a few things we offer:
- The best trading Platform
- No Requotes
- Lowest Spreads
- High-level liquidity
- Interbank connectivity
- Pure STP/DMA/ECN
- Free signals
- Best support
- Crypto Wallet and withdrawals / Deposits (USDT)
- Robust CRM
- TradeFxP wallet
- Once click withdrawal
- Multiple payment options
- Local offices to walk in
- Free VPS
- Free Video Chat / Virtual Meetings
- And many more…
If you choose to be a part of our managed account program:
- All of the above +
- 1-2% Daily Profits
- High-level risk management
- Capital protection
- Only 30% of the capital used
- Negative balance protection
- Our fee is from the profits only
- Monthly profit withdrawal
- Wallet system – Use it like Phonepe, or Google Pay
- Crypto wallet and withdrawals / Deposits (USDT)
- Live monitoring
- MyFxbook Live monitoring
- Copy Trading
- And many more…
Optional: If you do not withdraw your profits for 2 months, our system will use those profits to trade and will keep your 100% capital safe and secure for margin purposes. This is optional, and if you choose not to be a part of it, you can withdraw your profits from the first month itself.
Why 1-2% daily? Can't your managed forex account earn more?
Yes, we can! Remember: greed may be good in the beginning, but in the end, it will destroy everything. You and I know that! Many droplets make an ocean! Join the Managed Account Program and sit back for six months, then look at your account. You'll see that our strategy is good and the best. Do you know what I mean?
If you choose to be a part of us as an introducing broker (IB) or channel partner,
- Industry best Rebates
- Local Office support
- Staff support
- Marketing support
- Marketing materials
- And many more…
Having said that….
You can join our Forex Managed Account program and earn 1-2% profits daily. See for yourself by clicking the below link.
Have a great journey, and may you catch some big waves on your way to prosperity!
To see Ai Forex Trading for real, use these credentials.
- Low-risk strategy:
- Mt4: 112018
- Pw: Allah@101
- Server: tradefxp live,
1. To read why you should be with us, click here.
2. To open an account, click here.
3. To see our regulation certificate, click here.
4. To see our news with the IFMRRC, click here.
5. For claims, click here.
6. For the main site, click here.
7. For blogs and articles, click here.
8. Main Website: www.TradeFxP.com