The Impact of Algorithms: From Airport Security to University Admissions
"Explore the profound and wide-ranging impact of algorithms in 'From Airport Security to University Admissions.' This insightful article examines how algorithms are reshaping critical aspects of society, from enhancing airport security measures to influencing university admission processes."
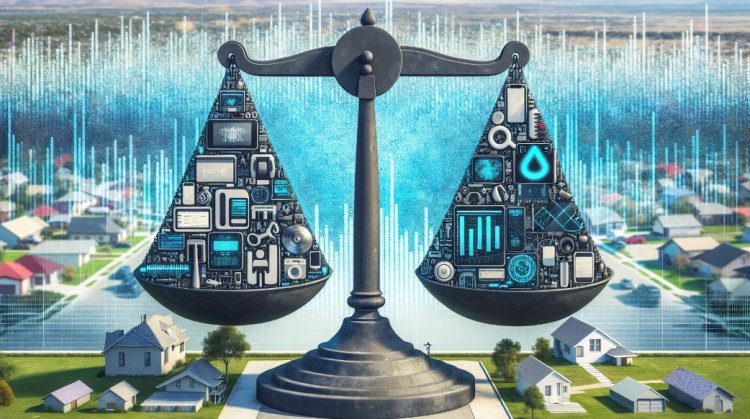
The Impact of Algorithms: From Airport Security to University Admissions
Introduction: Algorithms and Privilege
Algorithms are shaping our lives in ways we may not even realise. From airport security to university admissions, these algorithms have a profound impact on our experiences and opportunities. As someone who has personally experienced the consequences of algorithmic classification, I understand the importance of examining the role of algorithms in society.
Personal experience with airport security
Standing in the security line at the airport, I couldn't help but feel a mix of privilege and anxiety. As a white individual with US citizenship and an affiliation with a prestigious institution, I knew I had certain advantages. However, the moment I stepped into the millimetre wave scanning machine, I became acutely aware of the potential for algorithmic discrimination. The scanner's algorithm, based on normative body shapes, flagged my body as anomalous, leading to a humiliating search by security officers. This experience highlighted the ways in which algorithms can classify individuals based on appearance alone, perpetuating biases and reinforcing societal inequalities.
The consequences of algorithmic classification
Algorithmic classification has far-reaching consequences, impacting everything from personal security to access to education. In cases like airport security, individuals who deviate from normative body shapes or appearances can be disproportionately targeted and subjected to invasive searches. In other contexts, such as university admissions, algorithms determine which students are offered places based on undisclosed criteria. This lack of transparency can disadvantage students from marginalised backgrounds who may not have access to the information needed to navigate the system effectively.
The increasing role of AI in our lives
Artificial intelligence (AI) is becoming increasingly prevalent in our daily lives. We rely on AI-powered systems to make decisions that affect us, from determining our creditworthiness to recommending products and services. However, these systems are not infallible. They are built on algorithms that learn from data, including biassed and discriminatory data. As a result, AI systems can perpetuate and amplify existing inequalities, further entrenching privilege and disadvantage.
It is crucial that we critically examine the role of algorithms and AI in our society. By understanding the potential for bias and discrimination, we can work towards creating fairer and more equitable systems. In the following sections, we will explore real-world examples of algorithmic privilege and its consequences, shedding light on the need for greater transparency, accountability, and diversity in the development and deployment of these technologies.
Examining Algorithmic Systems: ImageNet Roulette
Algorithmic classification systems have become increasingly prevalent in our daily lives, shaping our experiences and opportunities. One notable example of such a system is ImageNet Roulette, an experiment designed to explore the biases and limitations of algorithmic classification.
Introduction to ImageNet Roulette
Trevor Paglen, a visual artist, and Kate Crawford, a researcher at Microsoft, created ImageNet Roulette as an online application. It utilises an algorithm trained on the ImageNet dataset, one of the largest image databases in the world, to classify uploaded images into different categories. The goal of ImageNet Roulette is to provide users with a glimpse into how an algorithmic system perceives and classifies individuals.
Experimenting with algorithmic classification
By uploading their own photos to ImageNet Roulette, users can see how the algorithm categorises them. The results can be surprising and sometimes absurd, as the algorithm's classification is based on patterns it has learned from the dataset. Users have reported being misclassified as professions, objects, or even labelled with negative attributes.
This experiment raises important questions about the accuracy and reliability of algorithmic classification systems. It highlights how these systems can make assumptions and judgements based on appearances alone without considering the complexity and nuances of individual identity.
The limitations and biases of algorithms
ImageNet Roulette serves as a powerful reminder of the limitations and biases inherent in algorithmic systems. Algorithms are not neutral or infallible; they are trained on data that can be biassed or discriminatory, leading to errors and perpetuating existing inequalities.
The misclassifications and biases exposed by ImageNet Roulette demonstrate the need for greater transparency, accountability, and diversity in the development and deployment of algorithmic systems. It is crucial that we critically examine these systems to ensure they are fair, equitable, and respectful of individual rights and identities.
Protecting against Domestic Violence: The VEN Algorithm
In the fight against domestic violence, Spain has implemented an algorithmic system called VEN (Violence Against Women). This system aims to evaluate the risk that gender-based violence victims face and forecast the likelihood of future attacks by offenders. While VEN is not intended to replace the police, it serves as a support tool for law enforcement in their decision-making process.
Case study: Macarena's experience
Macarena Garcia, a victim of domestic violence, shares her story of how VEN was utilised in her case. Despite enduring years of abuse, Macarena found it difficult to receive the necessary support and protection. When she finally sought help from the police, she was subjected to a series of questions, which were then evaluated by the VEN algorithm to determine her level of risk. Unfortunately, the algorithm classified Macarena's risk as medium, underestimating the severity of her situation.
Macarena's case highlights the limitations of algorithmic risk assessment. While VEN aims to provide objective evaluations, it may not always capture the full complexity of an individual's circumstances. In Macarena's case, the algorithm failed to accurately assess the imminent danger she faced, leading to inadequate protection measures.
Issues with algorithmic risk assessment
The use of algorithmic systems, like VEN, in risk assessment poses several challenges:
-
Lack of transparency: The exact criteria and methodology used by the algorithm are often undisclosed, making it difficult for individuals to understand how their risk is being evaluated.
-
Data biases: algorithms learn from historical data, which can be biassed and perpetuate existing inequalities. In the case of VEN, the algorithm's reliance on standardised questions may overlook crucial contextual factors that influence the level of risk.
-
Over-reliance on algorithms: Law enforcement officers and judges may place undue trust in the algorithm's assessment, leading to complacency and disregarding other forms of evidence or individual judgement.
It is essential to critically examine and address these issues to ensure that algorithmic systems like VEN are fair, effective, and accountable. Striking a balance between the use of technology and the expertise of human professionals is crucial to protecting victims of domestic violence and preventing further harm.
University Admissions and Algorithmic Selection
In the context of university admissions, algorithmic selection plays a significant role in determining which students are offered places. One example of this is the Parkour Soup system in France, which was introduced in 2018 as a supposedly fair and transparent alternative to the previous admissions process. However, concerns have been raised regarding the lack of transparency and the impact on students from marginalised backgrounds.
Introduction to Parkour Soup in France
Parkour Soup is an online tool used for allocating university places in France. With over 177,000 educational programmes available, the system aims to match students with their preferred universities based on their preferences. However, the complexity of the system and the undisclosed ranking criteria used by universities have raised questions about its fairness and transparency.
Intisar's experience with the algorithm
Intisar, a high school graduate with outstanding exam results, shares her experience with the Parkour Soup algorithm. Despite her achievements, she did not receive a university place and was left without any explanation. This lack of transparency in the selection process leaves students like Intisar feeling frustrated and discouraged, as they are left wondering what criteria universities are using to evaluate their applications.
Lack of transparency in university admissions
The lack of transparency in university admissions is a concerning issue. Students from marginalised backgrounds, who may already face barriers to accessing higher education, are further disadvantaged by the undisclosed criteria used by algorithms. Without access to this information, they are unable to navigate the system effectively and may miss out on opportunities for further education.
Furthermore, the reliance on algorithms to make decisions about students' futures raises questions about the fairness and accuracy of these systems. Students are left in the dark about how their applications are being evaluated and whether other factors, such as the reputation of their high school, are being considered.
It is crucial for universities and educational institutions to address these concerns and ensure greater transparency, accountability, and diversity in the development and deployment of algorithmic systems. By doing so, they can ensure that the admissions process is fair, equitable, and accessible for all students, regardless of their background or circumstances.
Eating disorders and social media algorithms
The influence of K-pop and social media on body image:
Social media algorithms, particularly those used on platforms like YouTube and Instagram, have a significant impact on body image. One example of this influence is the rise of K-pop and its associated beauty standards. K-pop idols are often portrayed as having slim and "perfect" bodies, which can lead to unrealistic expectations and body dissatisfaction among fans. The constant exposure to these images can create a filter bubble, where users are only shown content that reinforces these beauty ideals.
Marie's journey into an eating disorder:
Marie's story is a powerful example of how social media algorithms can contribute to the development of eating disorders. As Marie became more immersed in the world of K-pop and started seeing content related to dieting and weight loss, she began to internalise the belief that the only acceptable figure was a thin one. This led to her restricting her food intake and ultimately developing an eating disorder.
The addictive nature of social media algorithms:
Social media algorithms are designed to keep users engaged for as long as possible, and they can be highly addictive. Algorithms learn from user behaviour and show content that is most likely to grab their attention and keep them scrolling. In Marie's case, she found herself spending hours scrolling through food-related content without feeling the need to actually eat. This addictive nature of social media algorithms can exacerbate eating disorders and make it even harder for individuals to break free from unhealthy behaviours.
It is important to recognise the harmful impact that social media algorithms can have on body image and mental health. By understanding the influence of these algorithms and being mindful of the content we consume, we can take steps to protect ourselves and promote a healthier relationship with our bodies.
Tax Scandals and Algorithmic Discrimination
In recent years, algorithmic systems have come under scrutiny for their potential to perpetuate discrimination and bias. One notable example of algorithmic discrimination is the Dutch tax benefit scandal, which has had a devastating impact on families across the country.
Overview of the Dutch tax benefit scandal
The Dutch tax benefit scandal involved the use of an algorithmic risk management system by the tax authorities to identify potential fraudsters in the child care benefits program. Non-Dutch nationals and those with dual citizenship were automatically flagged as potential fraudsters, leading to repayment demands and allegations of fraudulent claims. However, many of these allegations were false, and innocent families were forced into financial hardship.
Discrimination against non-Dutch nationals
The algorithm used in the tax benefit scandal unfairly targeted non-Dutch nationals, leading to a disproportionate number of them being falsely accused of fraud. This discrimination not only violated their rights but also perpetuated harmful stereotypes and biases against certain ethnic groups.
The devastating impact on families
The consequences of the tax benefit scandal were devastating for many families. Innocent parents were burdened with repayment demands they could not afford, leading to financial ruin and even homelessness. Children were removed from their families and placed in social care, causing immense emotional distress and trauma.
This scandal highlights the dangers of relying solely on algorithmic systems without proper oversight and accountability. The algorithm used in this case had serious flaws and led to widespread discrimination and injustice. It is crucial that we critically examine and address these issues to ensure fair and equitable systems that do not harm vulnerable individuals and communities.
Responsibility and Accountability in Algorithmic Systems
As algorithmic systems continue to shape our lives, it becomes crucial to examine who is responsible for the decisions made by these algorithms. The consequences of algorithmic classification can have far-reaching implications, from personal security to access to education. It is important to establish transparency and regulation to ensure that these systems are fair, accountable, and unbiased.
Examining who is responsible for algorithmic decisions
Algorithmic decisions are not made in a vacuum. People with the ability to influence the results are those who create and program them. The responsibility lies with the developers and programmers who design the algorithms and determine how they classify individuals. It is essential for these individuals to be aware of the potential biases and discriminatory impacts of their algorithms.
The need for transparency and regulation
Transparency is key to holding algorithmic systems accountable. Users and individuals affected by these systems should have access to information about how the algorithms work, what data they rely on, and how they make decisions. This transparency allows for scrutiny and evaluation, ensuring that these systems are fair and unbiased.
Regulation is also necessary to establish guidelines and standards for algorithmic systems. There should be clear guidelines on how these systems should be developed, tested, and deployed, with a focus on fairness and ethical considerations. Regulation can help prevent misuse and ensure that these systems do not perpetuate existing inequalities or discriminate against certain individuals or groups.
The social and ethical implications of algorithms
Algorithms have significant social and ethical implications. They have the potential to perpetuate biases, reinforce inequalities, and impact individuals' opportunities and experiences. It is crucial to critically examine these implications and work towards creating algorithms that are fair, equitable, and respectful of individual rights and identities.
By addressing responsibility, transparency, and regulation in algorithmic systems, we can strive towards creating a more equitable and just society. It is essential for developers, regulators, and society as a whole to actively engage in the conversation and take action to ensure that algorithmic systems are held accountable and serve the best interests of all individuals.